LiDar point cloud data morphological filtering method based on area prediction
A technology of morphological filtering and point cloud data, which is applied in image data processing, instruments, calculations, etc., can solve the problems of weak self-adaptation and achieve good filtering results, high efficiency, and fast speed
- Summary
- Abstract
- Description
- Claims
- Application Information
AI Technical Summary
Problems solved by technology
Method used
Image
Examples
Embodiment 1
[0057] In this embodiment, the method of the present invention is used to perform filtering simulation on the test sample sample 12 provided by ISPRS (International Society for Photogrammetry and Remote Sensing).
[0058] The simulation conditions are carried out under MATLAB2010a software.
[0059] Reference figure 1 , Perform a simulation experiment on the test sample sample12, which contains 52119 points of point cloud data. figure 1 (a) is the initial surface model (DSM), which reflects the original topography of the experimental area; figure 1 (b) Display the distribution of the ground point cloud in the sample data, all blanks are the ground feature points that should be filtered out; figure 1 (c) is the distribution result of the ground point cloud after filtering by this algorithm; figure 1 (d) is the final digital elevation model (DEM).
[0060] From figure 1 (a) It can be seen that the data feature of samp12 is a mixture of buildings and vegetation on the hillside, and...
Embodiment 2
[0062] In this embodiment, the method of the present invention is used to simulate the filtering of the test sample sample31 provided by ISPRS.
[0063] Simulation 2 simulation conditions are carried out under MATLAB2010a software.
[0064] Reference figure 2 , Perform simulation experiments on the test sample sample31, which contains 28862 points of point cloud data.
[0065] figure 2 (a) is DSM, reflecting the original topography of the experimental area; figure 2 (b) Display the distribution of the ground point cloud in the sample data, all blanks are the ground feature points that should be filtered out; figure 2 (c) is the distribution result of the ground point cloud after filtering in the present invention; figure 2 (d) is the final DEM generated.
[0066] From figure 2 (a) It can be seen that sample31 is mainly discontinuous terrain and low points, including complex buildings, vegetation and other targets. From figure 2 (c) and figure 2 (b) It can be seen that the effe...
Embodiment 3
[0068] In this embodiment, the method of the present invention is used to simulate the filtering of the test sample sample53 provided by ISPRS.
[0069] Simulation 3 simulation conditions are carried out under MATLAB2010a software.
[0070] Reference image 3 , Perform simulation experiments on the test sample sample53, which contains 34378 points of point cloud data. image 3 (a) is DSM, reflecting the original topography of the experimental area; image 3 (b) Display the distribution of the ground point cloud in the sample data, all blanks are the ground feature points that should be filtered out; image 3 (c) is the distribution result of the ground point cloud after filtering by this algorithm; image 3 (d) is the final DEM generated.
[0071] From image 3 (a) It can be seen that the data of samp53 is mainly discontinuous terrain with few buildings, but its data structure is more complicated, the discontinuous steep slopes are separated by layers, and buildings are distributed on...
PUM
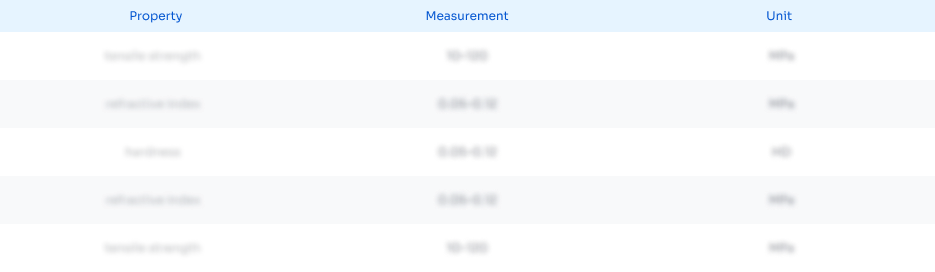
Abstract
Description
Claims
Application Information
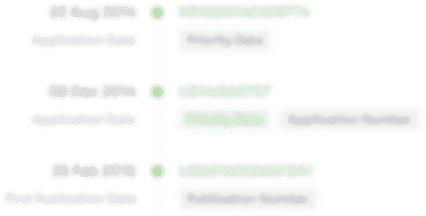
- R&D Engineer
- R&D Manager
- IP Professional
- Industry Leading Data Capabilities
- Powerful AI technology
- Patent DNA Extraction
Browse by: Latest US Patents, China's latest patents, Technical Efficacy Thesaurus, Application Domain, Technology Topic, Popular Technical Reports.
© 2024 PatSnap. All rights reserved.Legal|Privacy policy|Modern Slavery Act Transparency Statement|Sitemap|About US| Contact US: help@patsnap.com