Method for remain useful life prognostic of lithium ion battery with model active updating strategy
A lithium-ion battery, life prediction technology, applied in the direction of measuring electricity, measuring devices, measuring electrical variables, etc., can solve the problems of poor prediction accuracy, model mismatch can not be adjusted adaptively, achieve flexible non-parametric inference, overcome energy regeneration predicting difficult effects
- Summary
- Abstract
- Description
- Claims
- Application Information
AI Technical Summary
Problems solved by technology
Method used
Image
Examples
specific Embodiment approach 2
[0083] Embodiment 2. The difference between this embodiment and the method for predicting the remaining life of a lithium-ion battery with an active model update strategy described in Embodiment 1 is that the training data of the voltage and capacity of the lithium-ion battery are selected in step 1, and The specific process of constructing the initial SOH index from this data set is as follows:
[0084] Select the k-cycle data sequence of lithium-ion battery capacity and discharge voltage data from the beginning of battery use to the current charge-discharge cycle as the initial data sequence X(0)={x(0),x(1),...,x( k-1)}, where X(0) represents the data sequence obtained from the initial construction.
specific Embodiment approach 3
[0085] Specific Embodiment 3. The difference between this embodiment and the method for predicting the remaining life of a lithium-ion battery with an active model update strategy described in Embodiment 2 is that the sampling entropy feature extraction of the original data described in step 2, and the Gaussian process The specific process of regression to establish SOH index is as follows:
[0086] Step 31. Select the lithium-ion discharge voltage data sequence X of the charge-discharge cycle before the current moment obtained in step 1. 2 , construct N-m+1-dimensional vector x m (i)=[x(i), x(i+1),..., x(i+m-1)], i=[1, 2,..., N-m+1]. m is a value related to the battery degradation model. In this example, m=2, and the current moment is the corresponding moment of the latest data predicted by the rolling time window.
[0087] Step 32. Calculate the distance between two different discharge cycles according to the following formula:
[0088] d m [x m (i), x m (j)]=max[x m ...
specific Embodiment approach 4
[0110] Embodiment 4. The difference between this embodiment and the method for predicting the remaining life of lithium-ion batteries based on the model active update strategy described in Embodiment 1 or Embodiment 3 is that the specific method for judging whether the SOH prediction described in step 4 is completed The process is:
[0111] Use Gaussian process regression for multi-step forecasting, initially set n=5, and get the variance confidence range s of each step forecast i , according to s i Whether it is greater than twice the mean value of the confidence range of the previous i-1 step, that is, the following discriminant formula
[0112] s i ≥ 2 · Σ n = 0 i - 1 s ...
PUM
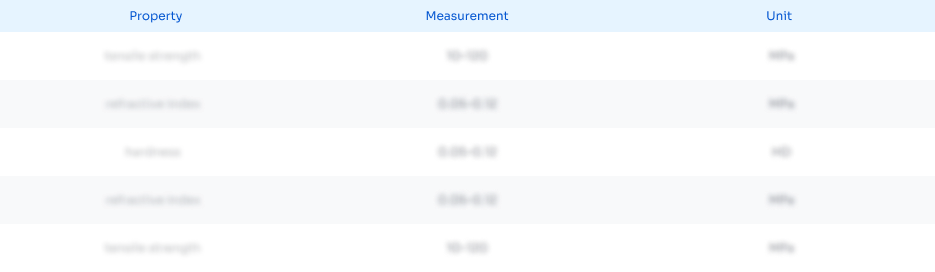
Abstract
Description
Claims
Application Information
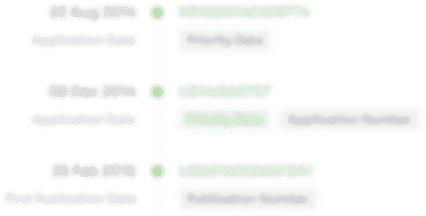
- R&D
- Intellectual Property
- Life Sciences
- Materials
- Tech Scout
- Unparalleled Data Quality
- Higher Quality Content
- 60% Fewer Hallucinations
Browse by: Latest US Patents, China's latest patents, Technical Efficacy Thesaurus, Application Domain, Technology Topic, Popular Technical Reports.
© 2025 PatSnap. All rights reserved.Legal|Privacy policy|Modern Slavery Act Transparency Statement|Sitemap|About US| Contact US: help@patsnap.com