A Method for Sparse Estimation of Remote Sensing Image Based on Hybrid Transformation
A remote sensing image and hybrid transformation technology, which is applied in the field of remote sensing image processing, can solve the problems of loss of image detail information, difficulty in obtaining better sparse effect of remote sensing image, etc., and achieve good sparse effect
- Summary
- Abstract
- Description
- Claims
- Application Information
AI Technical Summary
Problems solved by technology
Method used
Image
Examples
specific Embodiment approach 1
[0017] Specific Embodiment 1: A method for sparse estimation of remote sensing images based on hybrid transformation described in this embodiment, a method for sparse estimation of remote sensing images based on hybrid transformation, the method is implemented according to the following steps:
[0018] Step 1. Sparsely estimate the low frequency of the remote sensing image:
[0019] Step 1 (1), perform tensor product wavelet transform on the original image; Step 1 (2), use p-fold extraction filter to perform polyphase decomposition on each subband; Step 1 (3), perform decomposed component Principal component transformation; step 1 (4), retaining N for the transformed image 1 a larger transformation coefficient, and set the remaining coefficients to 0; get the result of sparse estimation, and inverse transform the above result;
[0020] Step 2. Sparsely estimate the high frequency of the remote sensing image:
[0021] Step 2 (1), subtract the original image from the low-frequ...
specific Embodiment approach 2
[0023] Embodiment 2: The difference between this embodiment and Embodiment 1 is that the sparse process of the low-frequency part of the remote sensing image is as follows: the original image is
[0024] The specific implementation process of step 1 (1) is as follows: Let A represent the two-dimensional tensor product wavelet transform, and the result of X after the tensor product wavelet transform represents XA T , the transformed image is expressed as:
[0025] XA T :=[(XA T ) (1) ,(XA T ) (2) ,…,(XA T ) (N) ]
[0026] In the formula, ":=" means "defined as", and N means the number of wavelet subbands;
[0027] The specific implementation process of step one (two) is: for each sub-band (XA T ) (i) The wavelet coefficients of the p-fold filter are used to generate transformation components, where i=1,...,N; the process is expressed as: symbol" "Indicates that after the variable before the sign is processed, it can be expressed as the variable after the sign; p...
specific Embodiment approach 3
[0035] Embodiment 3: The difference between this embodiment and Embodiment 1 or 2 is that after Step 1 (4) is completed, Step 1 (5) is performed: the estimation of the low-frequency part of the remote sensing image is carried out according to the following steps of:
[0036] Step 1. Apply principal component inverse transformation to each transformed component sequence Among them, i=1,2,...,N, the process is expressed as:
[0037] [ B ( 1 ) - 1 Y ~ ( 1 ) , B ( 2 ) - 1 Y ~ ( ...
PUM
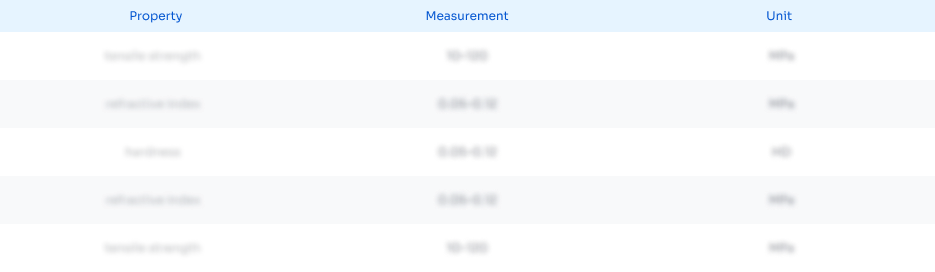
Abstract
Description
Claims
Application Information
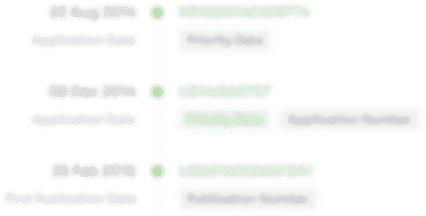
- Generate Ideas
- Intellectual Property
- Life Sciences
- Materials
- Tech Scout
- Unparalleled Data Quality
- Higher Quality Content
- 60% Fewer Hallucinations
Browse by: Latest US Patents, China's latest patents, Technical Efficacy Thesaurus, Application Domain, Technology Topic, Popular Technical Reports.
© 2025 PatSnap. All rights reserved.Legal|Privacy policy|Modern Slavery Act Transparency Statement|Sitemap|About US| Contact US: help@patsnap.com