SAR image terrain classification method based on depth RBF network
A technology of RBF network and ground object classification, which is applied to biological neural network models, instruments, character and pattern recognition, etc., can solve problems such as unstable results, slow speed, and difficult adjustment of classifier parameters, so as to reduce time complexity, The number of samples is reduced to avoid the effect of gradient diffusion
- Summary
- Abstract
- Description
- Claims
- Application Information
AI Technical Summary
Problems solved by technology
Method used
Image
Examples
Embodiment Construction
[0035] refer to figure 1 , the implementation steps of the present invention are described in detail as follows:
[0036] Step 1, given a deep RBF network composed of three layers.
[0037] Such as figure 2 As shown, the deep RBF network given in this example consists of a three-layer structure, in which the first layer and the third layer are RBF neural networks composed of an input unit, a hidden unit and an output unit; the second layer is A sparse autoencoder SAE neural network consisting of an input unit, a hidden unit and an output unit.
[0038] Step 2, preprocessing the SAR image, using the deep RBF network to learn the classification features of the SAR image.
[0039] (2a) Select SAR images containing towns, farmlands, and mountains as classification objects. Each type of ground object has 50 training samples and 100 test samples. The resolution of each sample is 128*128. Each sample is divided into image blocks with a resolution of 64*64, and 200 training sampl...
PUM
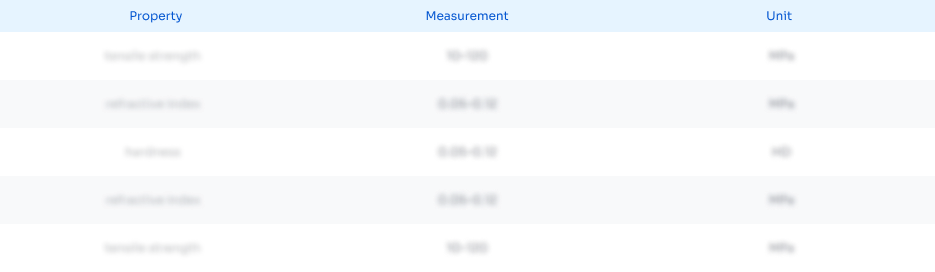
Abstract
Description
Claims
Application Information
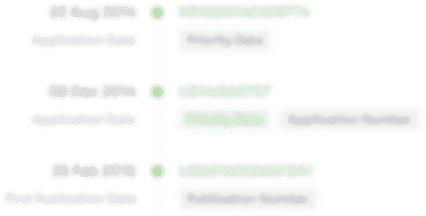
- R&D Engineer
- R&D Manager
- IP Professional
- Industry Leading Data Capabilities
- Powerful AI technology
- Patent DNA Extraction
Browse by: Latest US Patents, China's latest patents, Technical Efficacy Thesaurus, Application Domain, Technology Topic, Popular Technical Reports.
© 2024 PatSnap. All rights reserved.Legal|Privacy policy|Modern Slavery Act Transparency Statement|Sitemap|About US| Contact US: help@patsnap.com