Speaker recognition method based on depth learning
A speaker recognition and deep learning technology, applied in the field of speech processing, can solve the problems of unable to fully characterize the characteristics of the speaker's vocal tract, unable to automatically learn feature information, and unsatisfactory recognition effect, so as to improve the system recognition rate and reduce computational complexity. degree, the effect of a good classification function
- Summary
- Abstract
- Description
- Claims
- Application Information
AI Technical Summary
Problems solved by technology
Method used
Image
Examples
Embodiment
[0146] In the experiment, the parameters used are: voice sampling rate 16kHz, 16-bit coded PCM format voice, frame length 16 milliseconds, pre-emphasis coefficient a=0.9375; short-term energy and short-term zero-crossing rate thresholds are 67108864 and 30; select 10 speakers, the speech length of each speaker is about 10 seconds for training, and the speech unit length for testing is respectively 0.4 seconds, 0.8 seconds, 1.2 seconds, 1.6 seconds and 2.0 seconds, and the speech feature parameters Select 16-dimensional MFCC, 16-dimensional GFCC, and combine MFCC and GFCC to form a 32-dimensional feature vector. The number of hidden layers in the deep belief network model is 3 layers, and the number of neurons in each hidden layer is 50. , and the training times are 500 times. The speaker recognition results are shown in Table 3, and then the system recognition results of different speech features are drawn into a line graph as Figure 9 shown.
[0147] Table 3 Speaker recogn...
PUM
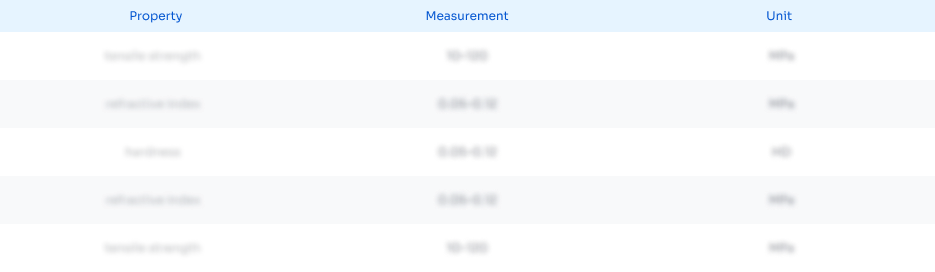
Abstract
Description
Claims
Application Information
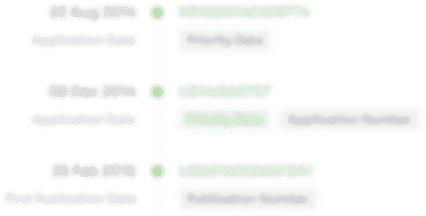
- R&D Engineer
- R&D Manager
- IP Professional
- Industry Leading Data Capabilities
- Powerful AI technology
- Patent DNA Extraction
Browse by: Latest US Patents, China's latest patents, Technical Efficacy Thesaurus, Application Domain, Technology Topic, Popular Technical Reports.
© 2024 PatSnap. All rights reserved.Legal|Privacy policy|Modern Slavery Act Transparency Statement|Sitemap|About US| Contact US: help@patsnap.com