Bearing variable-condition fault diagnosis method based on LMD-SVD (Local Mean Decomposition-Singular Value Decomposition) and extreme learning machine
An extreme learning machine and fault diagnosis technology, applied in mechanical bearing testing, special data processing applications, instruments, etc., can solve the problems of increasing SVM training time, difficult to select multiple parameters, easy to fall into local extreme values, etc., to avoid preset The effect of many parameters, fast learning speed, and reduced complexity
- Summary
- Abstract
- Description
- Claims
- Application Information
AI Technical Summary
Problems solved by technology
Method used
Image
Examples
Embodiment Construction
[0029] The present invention will be further described below in conjunction with the accompanying drawings and specific embodiments.
[0030] A kind of fault diagnosis method based on local mean decomposition (local mean decomposition, LMD), singular value decomposition (singular value decomposition, SVD) and extreme learning machine (extreme learning machine, ELM) of the present invention, concrete steps are as follows:
[0031] 1. Local Mean Decomposition
[0032]Local mean decomposition (local mean decomposition, LMD) is a new adaptive time-frequency analysis method proposed by Smith and 2005, which can adaptively decompose nonlinear and non-stationary vibration signals into a series of product functions (product functions) ,PFs), where each PF is the product of an envelope signal and a pure FM signal with instantaneous physical meaning, the envelope signal is the instantaneous amplitude of the PF component, and the PF component can be obtained by using the pure FM signal ...
PUM
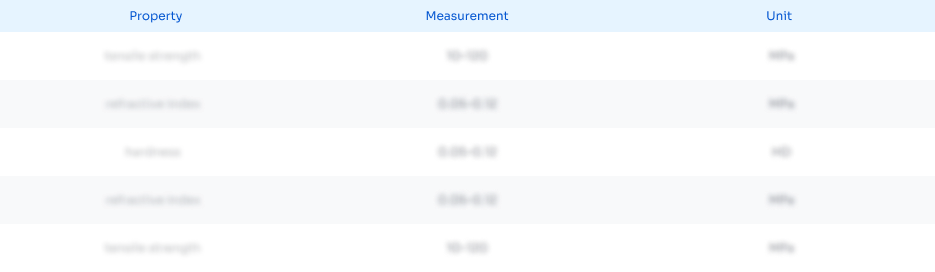
Abstract
Description
Claims
Application Information
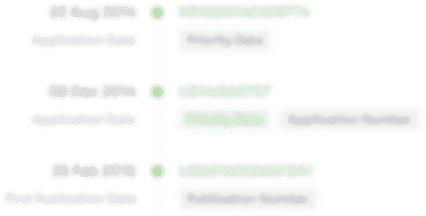
- R&D
- Intellectual Property
- Life Sciences
- Materials
- Tech Scout
- Unparalleled Data Quality
- Higher Quality Content
- 60% Fewer Hallucinations
Browse by: Latest US Patents, China's latest patents, Technical Efficacy Thesaurus, Application Domain, Technology Topic, Popular Technical Reports.
© 2025 PatSnap. All rights reserved.Legal|Privacy policy|Modern Slavery Act Transparency Statement|Sitemap|About US| Contact US: help@patsnap.com