Short-term load predicting method of power grid
A short-term load forecasting and power grid technology, applied in forecasting, instrumentation, data processing applications, etc., can solve the problems of unresolved prematurity, premature convergence, and affecting practicality, etc.
- Summary
- Abstract
- Description
- Claims
- Application Information
AI Technical Summary
Problems solved by technology
Method used
Image
Examples
Embodiment Construction
[0072] The technical solution of the present invention will be described in detail below in conjunction with the accompanying drawings and specific embodiments, so as to understand the essence of the present invention more clearly and intuitively.
[0073] Such as figure 1 As shown, the grid short-term load forecasting method proposed by the present invention includes the following steps:
[0074] Step 1, obtain historical data and preprocess the data;
[0075] In the step S1, the historical data includes load data and weather data of the past two years. Among them, the time resolution of the load data is 5 minutes, that is, there are 288 data samples in one day. The sample data is preprocessed, the time resolution of the data is changed to 1h, and the average power per hour is the power at that moment. Weather data includes maximum and minimum temperatures and rainfall for the past two years.
[0076] Step 2, using wavelet decomposition to decompose the historical load sa...
PUM
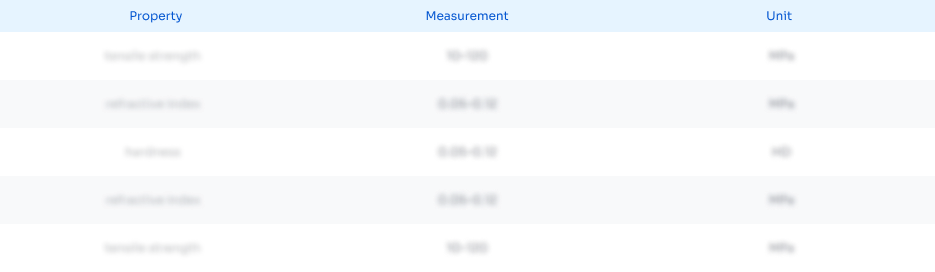
Abstract
Description
Claims
Application Information
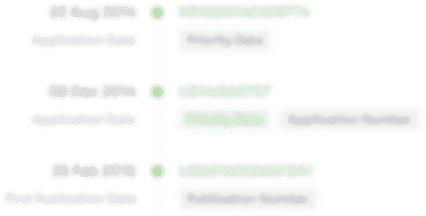
- R&D
- Intellectual Property
- Life Sciences
- Materials
- Tech Scout
- Unparalleled Data Quality
- Higher Quality Content
- 60% Fewer Hallucinations
Browse by: Latest US Patents, China's latest patents, Technical Efficacy Thesaurus, Application Domain, Technology Topic, Popular Technical Reports.
© 2025 PatSnap. All rights reserved.Legal|Privacy policy|Modern Slavery Act Transparency Statement|Sitemap|About US| Contact US: help@patsnap.com