EMD based fiber-optic gyroscope temperature drift multi-scale extreme learning machine training method
An extreme learning machine and temperature drift technology, applied in special data processing applications, instruments, electrical digital data processing, etc., can solve problems such as complex modeling process and limited ability to approach complex nonlinear
- Summary
- Abstract
- Description
- Claims
- Application Information
AI Technical Summary
Problems solved by technology
Method used
Image
Examples
Embodiment 1
[0038] This embodiment mainly includes the following steps:
[0039] Step 1: Use the BEEMD method to adaptively decompose the temperature drift data into a series of intrinsic mode functions (IMF), set the temperature drift data as x(t), and the order of noise assistance as M=m-1, add Gaussian white noise w j The degree of (t) is I, and the noise variance is where k is the current decomposed IMF order, which is initially 1, and j represents the count of noise-assisted realization, and the decomposition process is:
[0040] Initialize variable j=0,
[0041] add random white noise to which is h k j ( t ) = h k j ( t ) + β k E k ( w j ...
PUM
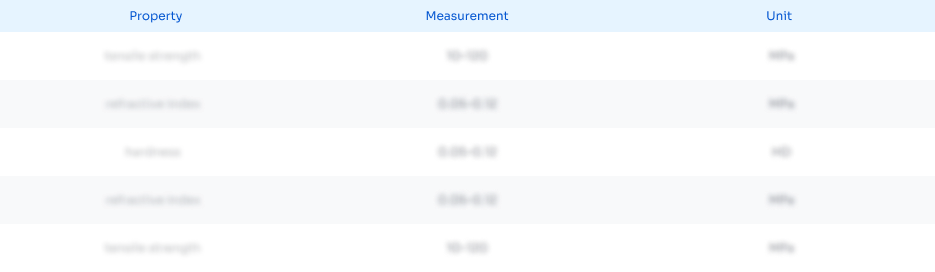
Abstract
Description
Claims
Application Information
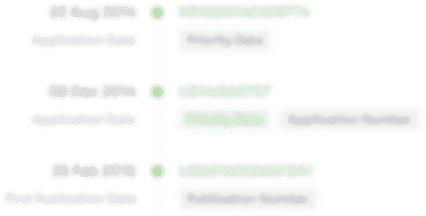
- R&D
- Intellectual Property
- Life Sciences
- Materials
- Tech Scout
- Unparalleled Data Quality
- Higher Quality Content
- 60% Fewer Hallucinations
Browse by: Latest US Patents, China's latest patents, Technical Efficacy Thesaurus, Application Domain, Technology Topic, Popular Technical Reports.
© 2025 PatSnap. All rights reserved.Legal|Privacy policy|Modern Slavery Act Transparency Statement|Sitemap|About US| Contact US: help@patsnap.com