A Remote Sensing Quantitative Inversion Method for Sampling Learning Machine Adapted to Noise Conditions
A learning machine and inversion technology, applied in the field of remote sensing applications, can solve the problems of nonlinear noise and interference in the quantitative inversion of remote sensing, and achieve the effect of strong generalization ability, eliminating the influence of noise, and improving accuracy.
- Summary
- Abstract
- Description
- Claims
- Application Information
AI Technical Summary
Problems solved by technology
Method used
Image
Examples
Embodiment Construction
[0016] As a nonlinear and statistical modeling tool, artificial neural network (ANN) is widely used in remote sensing quantitative inversion. The model can fully approximate any complex nonlinear relationship through the setting of neural structure and connection weights. However, due to the defects of the ANN model itself, such as slow learning convergence, easy to fall into local extremum, and difficult to determine the network structure, the inversion accuracy is difficult to meet the application requirements. Huang et al. improved the traditional ANN model and proposed an extreme learning machine (Extreme Learning Machine, ELM) algorithm. The ELM algorithm is a new type of single hidden layer feed-forward neural network, and its learning speed and generalization ability are greatly improved compared with the ANN model. This mainly depends on two aspects of improvement: (1) Randomly generate a small order of magnitude value as the connection weight between the input layer ...
PUM
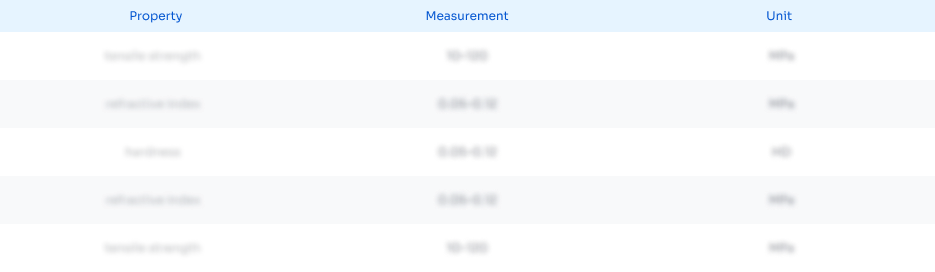
Abstract
Description
Claims
Application Information
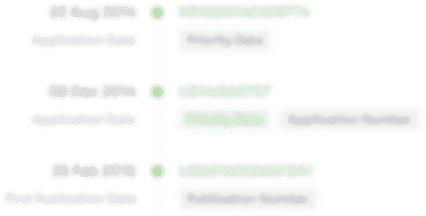
- R&D Engineer
- R&D Manager
- IP Professional
- Industry Leading Data Capabilities
- Powerful AI technology
- Patent DNA Extraction
Browse by: Latest US Patents, China's latest patents, Technical Efficacy Thesaurus, Application Domain, Technology Topic.
© 2024 PatSnap. All rights reserved.Legal|Privacy policy|Modern Slavery Act Transparency Statement|Sitemap