Multi-modal process fault detection method and system based on vine copula correlation description
A fault detection and correlation technology, applied in general control systems, control/regulation systems, simulators, etc., to solve problems such as the complexity of the parameter optimization process
- Summary
- Abstract
- Description
- Claims
- Application Information
AI Technical Summary
Problems solved by technology
Method used
Image
Examples
Embodiment 1
[0076] see image 3 , the present invention discloses a multimodal chemical process fault detection method based on vine copula correlation modeling and Bayesian inference, the specific steps are as follows:
[0077] [Step S1] Obtain training sample sets of normal data in different modalities according to expert knowledge or clustering method.
[0078] [Step S2] Carry out correlation modeling by using C-vine copula to obtain the joint probability density function of each mode.
[0079] For n-dimensional random vector x=[x 1 ,x 2 ,...,x n ] T , its C-vine model (joint probability density function of x) is:
[0080] f ( x ) = Π t = 1 n f t ( x t ) × Π i ...
Embodiment 2
[0122] The description of the following examples will help to understand the present invention, but does not limit the content of the present invention. see figure 2 , this embodiment realizes the multi-modal fault detection of the TE process in the case of mode one and mode three, and the production load and other related parameters of the two modes are shown in Table 1. The TE process studied in this example is a steady-state process under closed-loop control, and the process data are collected from 22 common process variables, and the sampling time is set to 0.05h. 1000 sets of training sample data are obtained from mode 1 and mode 3. The first 200 sets of data of the test sample data come from mode 3, and the last 200 sets of data come from mode 1, where fault 13 (drift) occurs from the 101st to 200th time, and fault 6 (drift) occurs from the 301st to 400th time step).
[0123] Table 1: Parameter settings of TE process mode 1 and mode 3
[0124]
[0125] (1) Accord...
Embodiment 3
[0137] A multimodal process fault detection method based on vine copula correlation description, said method comprising the steps of:
[0138] Step S1, obtaining training sample sets of normal data in different modalities;
[0139] Step S2, performing correlation modeling to obtain the joint probability density function of each mode;
[0140] Step S3, sampling the joint probability density function of different modes, and calculating the joint probability density function value of each sample;
[0141] Step S4, determine the discretization step size l according to the control limit, and use the density quantile method to construct the static density quantile table of the process;
[0142] Step S5, estimate the monitoring data at time t by means of table lookup Generalized local probability index under mode k P L ( k ) ( X t ...
PUM
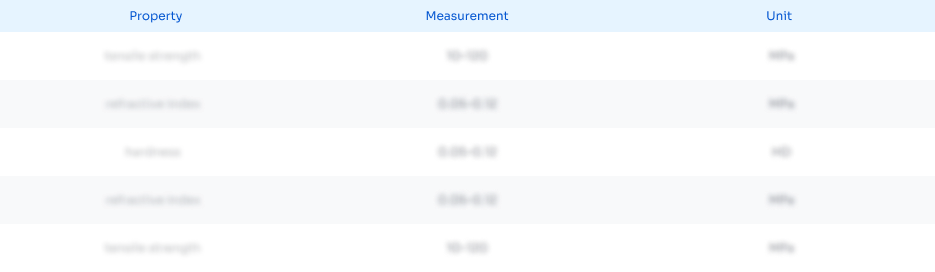
Abstract
Description
Claims
Application Information
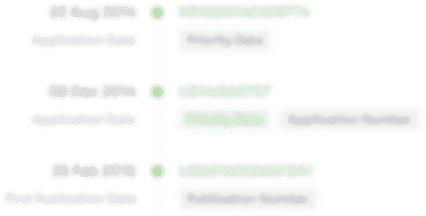
- R&D Engineer
- R&D Manager
- IP Professional
- Industry Leading Data Capabilities
- Powerful AI technology
- Patent DNA Extraction
Browse by: Latest US Patents, China's latest patents, Technical Efficacy Thesaurus, Application Domain, Technology Topic.
© 2024 PatSnap. All rights reserved.Legal|Privacy policy|Modern Slavery Act Transparency Statement|Sitemap