Training method of classifier, image detection method and respective system
A training method and strong classifier technology, applied in the field of image processing, can solve the problems of high classifier training cost, high classifier image detection error rate, and low classification training accuracy.
- Summary
- Abstract
- Description
- Claims
- Application Information
AI Technical Summary
Problems solved by technology
Method used
Image
Examples
Embodiment 1
[0045] Such as figure 1 As shown, the present invention provides a training method for a strong classifier. The training method is mainly performed by a training system. The training system is installed in computer equipment. The computer device can also be used for bad block detection based on 3D left and right views. The training samples received by the training system are pre-selected according to the needs of image detection. Each sample contains at least one type of eigenvalues. The feature value types include but are not limited to: difference feature type, SAD feature type, maximum difference feature type, and the like.
[0046] The purpose of the training method is to train a strong classifier with the smallest error probability for a single classification. In order to effectively classify the difference estimation blocks in the image during image detection.
[0047] The training method includes steps S11, S12 and S13. Wherein, the strong classifier is composed ...
Embodiment 2
[0085] In order to train classifiers that can be used for image detection, the present invention also provides a training method for cascade classifiers. Such as Figure 5 shown. The cascaded classifier is composed of several stages of strong classifiers as described in any one of the first embodiment in series.
[0086] Before training the above-mentioned cascade classifier, the training system can use various feature extraction methods to extract the feature values of the sample images (referred to as samples), and after each sample is formed into a sample space, the training system will use the preset samples Each sample in the space is filtered one by one through each order of strong classification.
[0087] Specifically, the training system trains the weak classifiers in the current strong classifier according to the samples received by the current strong classifier, and classifies the weak classifiers in the current strong classifier, The samples in the category wit...
Embodiment 3
[0092] The invention also provides an image detection method, which is used in a detection system of 3D left and right views. Here, the detection system is used to determine whether the corresponding image region is valid by detecting the feature values estimated by its own depth estimation module during the 3D image conversion process. The detection system is preset with cascaded strong classifiers trained through the aforementioned training steps. Wherein, at least two strong classifiers in the cascaded classifier classify the received difference estimation blocks into different classes according to their biased classes, such as Image 6 shown. The image detection method is specifically as follows:
[0093] In step S21, the detection system acquires feature values corresponding to a plurality of difference estimation blocks. Wherein, the feature value includes but not limited to: Disparity feature (Disparity), SAD feature, maximum difference difference feature, and th...
PUM
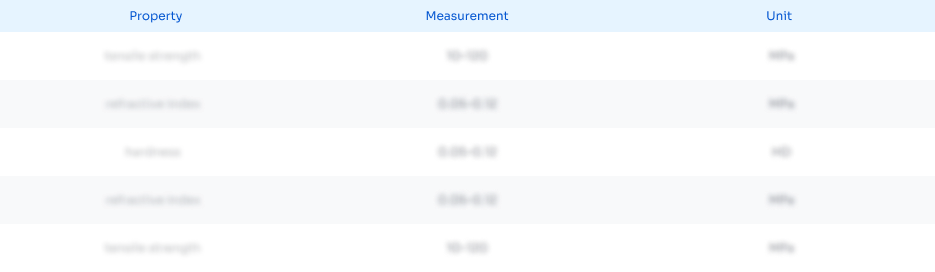
Abstract
Description
Claims
Application Information
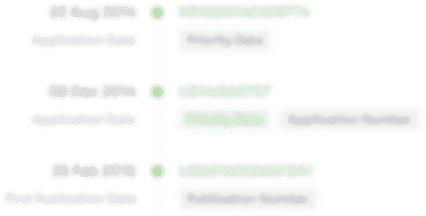
- R&D
- Intellectual Property
- Life Sciences
- Materials
- Tech Scout
- Unparalleled Data Quality
- Higher Quality Content
- 60% Fewer Hallucinations
Browse by: Latest US Patents, China's latest patents, Technical Efficacy Thesaurus, Application Domain, Technology Topic, Popular Technical Reports.
© 2025 PatSnap. All rights reserved.Legal|Privacy policy|Modern Slavery Act Transparency Statement|Sitemap|About US| Contact US: help@patsnap.com