ICA-PCA multi-working condition fault diagnosis method based on local neighborhood standardization and Bayesian inference
A local neighborhood and fault diagnosis technology, which is applied in the direction of instruments, electrical testing/monitoring, control/regulation systems, etc., can solve problems such as difficulty in obtaining prior knowledge, inconvenient observation and judgment, and difficulty in determining new data models
- Summary
- Abstract
- Description
- Claims
- Application Information
AI Technical Summary
Problems solved by technology
Method used
Image
Examples
Embodiment Construction
[0095] Combine below figure 1 Shown, the present invention is described in further detail:
[0096] Step 1: Collect the normal operation data of each working condition of the industrial process to form a training sample set.
[0097] Step 2: Use the local neighborhood normalization method to preprocess the training samples, so that the data of multiple working conditions can be represented by a single model. The local neighborhood normalization algorithm is:
[0098] Suppose the sample set X∈R m×n , where m is the number of process variables and n is the size of the sample data. sample x i ∈ R m×1 (i=1,2...,n) local neighborhood N k (x i ) represents the k nearest neighbors of the sample determined by the Euclidean distance in X, where Then use the neighborhood mean and neighborhood standard deviation of the first neighbor of each sample to perform standardization as in formula (1):
[0099] Z i = ...
PUM
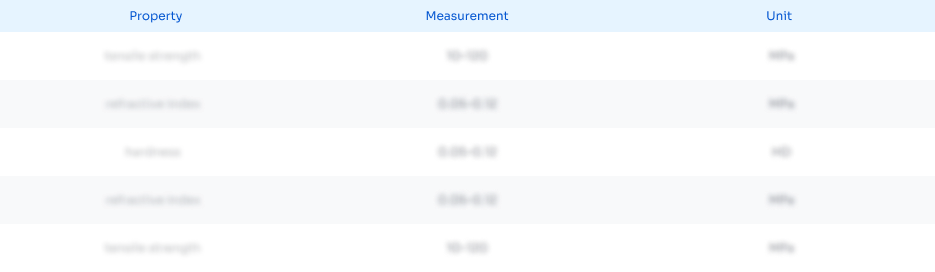
Abstract
Description
Claims
Application Information
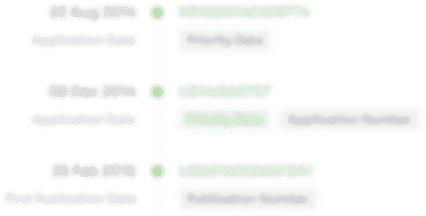
- R&D Engineer
- R&D Manager
- IP Professional
- Industry Leading Data Capabilities
- Powerful AI technology
- Patent DNA Extraction
Browse by: Latest US Patents, China's latest patents, Technical Efficacy Thesaurus, Application Domain, Technology Topic.
© 2024 PatSnap. All rights reserved.Legal|Privacy policy|Modern Slavery Act Transparency Statement|Sitemap