Chemical production on-line fault detection and diagnosis technique based on physical-large data hybrid model
A fault detection and hybrid model technology, applied in chemical data mining, electrical digital data processing, special data processing applications, etc., can solve problems such as the increase of fault diagnosis error rate and the mistrust of production technicians in fault detection.
- Summary
- Abstract
- Description
- Claims
- Application Information
AI Technical Summary
Problems solved by technology
Method used
Image
Examples
Embodiment 1
[0045] Embodiment 1: Detection and diagnosis of the stability failure of the system.
[0046] In order to test the effectiveness of the new method, the normal data and abnormal data sets are marked in the data set for testing, in which the normal data contains 61225 data points and 66 data points with stability failures (at 55370-55436 positions),
[0047] The first step is to use the real-time database of the device to collect data to form a training sample set for modeling: X R 61291×112 . Among them, the list is shown in Attached Table 1.
[0048] The second step targets production units with a defined physical model and extracts key performance indicators based on the physical model.
[0049] The feed heating furnace in the production process is taken as an example. For details, see figure 2 , in furnace unit Q, there are about 28 process variables, Xq R 61291×28 Some process variables are uncontrollable. For example, heat dissipation is usually related to the ins...
Embodiment 2
[0076] Embodiment 2: detection and diagnosis of the performance fault of this system:
[0077] Next, in conjunction with this specific process, the implementation steps of the present invention are described in detail:
[0078] The first step. Same as the first step in Example 1.
[0079] The second step; the same as the second step in Example 1.
[0080] The third step is to conduct a double-layer regression analysis on the performance approval standard to obtain a monitoring model for performance failure.
[0081] After extracting the key indicators of the physical model and reducing the dimensionality, Xnew containing relevant physical information is obtained and normalized (same example 1), and the relationship between Xnew and plant-level key performance indicators is regressed to monitor whether there is a performance failure in the process.
[0082] to attach figure 1Taking the production process in China as an example, the key performance indicators at the factory l...
PUM
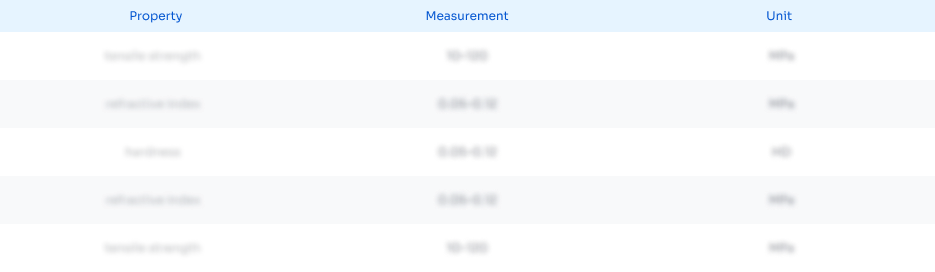
Abstract
Description
Claims
Application Information
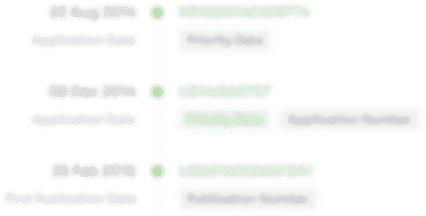
- R&D Engineer
- R&D Manager
- IP Professional
- Industry Leading Data Capabilities
- Powerful AI technology
- Patent DNA Extraction
Browse by: Latest US Patents, China's latest patents, Technical Efficacy Thesaurus, Application Domain, Technology Topic, Popular Technical Reports.
© 2024 PatSnap. All rights reserved.Legal|Privacy policy|Modern Slavery Act Transparency Statement|Sitemap|About US| Contact US: help@patsnap.com