A Remote Sensing Image Classification Method Based on Multimodal Deep Learning
A remote sensing image and deep learning technology, applied in the field of image processing, can solve the problem of low classification accuracy, achieve the effect of improving classification accuracy, reducing manual input, and simplifying complexity
- Summary
- Abstract
- Description
- Claims
- Application Information
AI Technical Summary
Problems solved by technology
Method used
Image
Examples
Embodiment Construction
[0031] The specific embodiments provided by the present invention will be described in detail below in conjunction with the accompanying drawings.
[0032] The present invention is a remote sensing image classification method based on multimodal deep learning, such as figure 1 shown, including the following steps:
[0033] Step 1: Construct a multi-modal sample set, and design the size of remote sensing images under different data modes according to different data modes;
[0034] The sample set includes different data modalities, and the sample size of each data modality is designed in different sizes according to different imaging principles such as its space and spectral resolution.
[0035] Specifically, for the same surface object to be classified, taking two data modalities as an example, the spatial resolutions of the two remote sensing images are h and h' respectively, and the sample size of the remote sensing image with resolution h is set to N×N, then The sample siz...
PUM
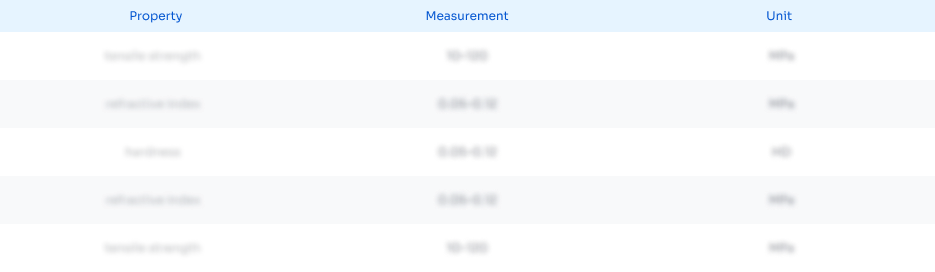
Abstract
Description
Claims
Application Information
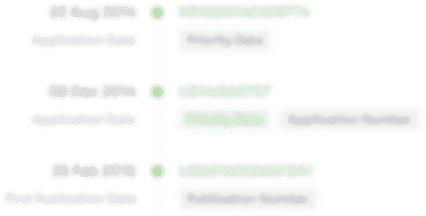
- R&D Engineer
- R&D Manager
- IP Professional
- Industry Leading Data Capabilities
- Powerful AI technology
- Patent DNA Extraction
Browse by: Latest US Patents, China's latest patents, Technical Efficacy Thesaurus, Application Domain, Technology Topic, Popular Technical Reports.
© 2024 PatSnap. All rights reserved.Legal|Privacy policy|Modern Slavery Act Transparency Statement|Sitemap|About US| Contact US: help@patsnap.com