Support vector regression machine model selection method
A technology of support vector regression and model selection, applied in computer parts, character and pattern recognition, instruments, etc., can solve problems such as occupying algorithm time
- Summary
- Abstract
- Description
- Claims
- Application Information
AI Technical Summary
Problems solved by technology
Method used
Image
Examples
Embodiment Construction
[0096] The present invention will be further described below in conjunction with accompanying drawing:
[0097] Such as figure 1 Shown: MKF-CKF-SVR model parameter selection
[0098] The selection method of the hyperparameters of the support vector regression model is deduced below, and the specific steps of the proposed algorithm are given. designed as figure 1 In the hyperparameter adjustment system shown, firstly, the original data set is divided into k groups by using the k-fold cross-validation method, the local kernel function and the global kernel function are selected to determine the mixed kernel function, and the k sub-LIBSVM is used to train the data set based on the mixed kernel function , and its predicted output is embedded into the volumetric Kalman filter, and the hyperparameters of the model are used as the state vector of the system, then the entire hyperparameter adjustment problem can be regarded as a filtering estimation problem of a nonlinear dynamic sy...
PUM
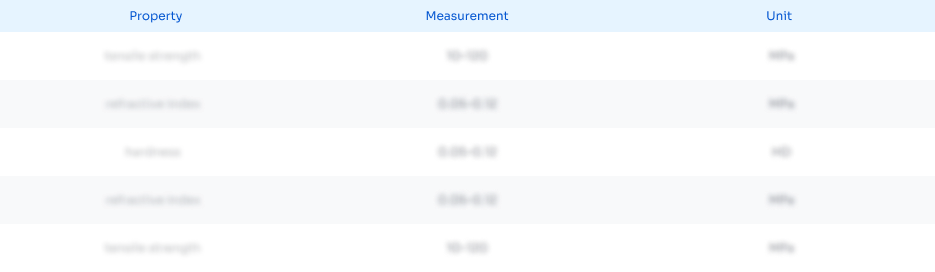
Abstract
Description
Claims
Application Information
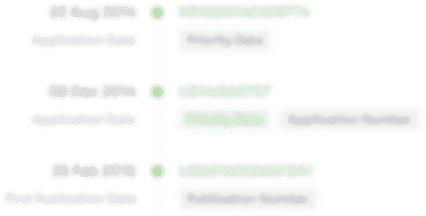
- R&D Engineer
- R&D Manager
- IP Professional
- Industry Leading Data Capabilities
- Powerful AI technology
- Patent DNA Extraction
Browse by: Latest US Patents, China's latest patents, Technical Efficacy Thesaurus, Application Domain, Technology Topic, Popular Technical Reports.
© 2024 PatSnap. All rights reserved.Legal|Privacy policy|Modern Slavery Act Transparency Statement|Sitemap|About US| Contact US: help@patsnap.com