Hyperspectral Remote Sensing Image Classification Method Based on Adaptive Hierarchical Multi-scale
A hyperspectral remote sensing and classification method technology, applied in the direction of instruments, scene recognition, calculation, etc., can solve the problems of high time cost, difficult to distinguish, limit mining of hyperspectral information, etc., to achieve strong global constraints, generalization ability to avoid, Avoid Time Complexity Effects
- Summary
- Abstract
- Description
- Claims
- Application Information
AI Technical Summary
Problems solved by technology
Method used
Image
Examples
Embodiment Construction
[0059] combine figure 1 , the present invention is based on an adaptive hierarchical multi-scale hyperspectral classification method, the specific process is:
[0060] Step 1, calculate the irregular neighborhood structure of the pixel according to the spectral angle;
[0061] for pixel x i , the coordinate position is (p i ,q i ), the initial square neighbor pixel area point position coordinates N(x i )for:
[0062]
[0063] In the formula, x is any point in the square area, the x coordinate position is (p, q), (2*M+1) is the side length of the square area;
[0064] This square neighborhood may have background points or may contain pixels of different categories. Thus, this neighborhood cannot correctly reflect the center pixel. In order to remove noise points, we calculate the spectral angle (SA, Spectral Angel) between the pixels in the area and the intermediate pixels, the formula is as follows:
[0065]
[0066] Select H-1 neighborhood pixels with the smalle...
PUM
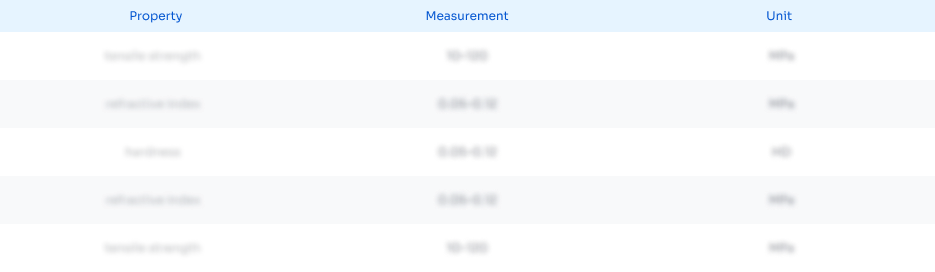
Abstract
Description
Claims
Application Information
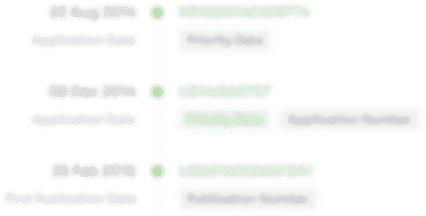
- R&D Engineer
- R&D Manager
- IP Professional
- Industry Leading Data Capabilities
- Powerful AI technology
- Patent DNA Extraction
Browse by: Latest US Patents, China's latest patents, Technical Efficacy Thesaurus, Application Domain, Technology Topic, Popular Technical Reports.
© 2024 PatSnap. All rights reserved.Legal|Privacy policy|Modern Slavery Act Transparency Statement|Sitemap|About US| Contact US: help@patsnap.com