Sparse and low-rank matrix approximation-based hyperspectral image restoration method
A hyperspectral image, low-rank matrix technology, applied in the field of hyperspectral image restoration, can solve the problems of ignoring hyperspectral image correlation and unsatisfactory effect.
- Summary
- Abstract
- Description
- Claims
- Application Information
AI Technical Summary
Problems solved by technology
Method used
Image
Examples
Embodiment Construction
[0069] Concrete implementation steps of the present invention include:
[0070] Step 1, acquire hyperspectral image data affected by mixed noise.
[0071] Use a hyperspectral imager to obtain a set of multi-band hyperspectral image data d, and normalize it to [0,1]. Its size is M×N×B, where M and N represent the length and width of the hyperspectral image of each band, respectively, and B represents how many bands there are in total. The estimated noise level for this set of data is η = 20 / 255.
[0072] Step 2, initialize iteration variables.
[0073] (2a) Let the denoised data noisy data Gaussian noise level η (0) = η.
[0074] (2b) Initialize the iteration, set the loop variable k=1, and the horizontal and vertical coordinates of the center are i=10, j=10 respectively.
[0075] Step 3, iterative regularization
[0076] Step 4, obtain the two-dimensional data of the low-rank model to be established.
[0077] (4a) For the M×N hyperspectral image of B bands, take o...
PUM
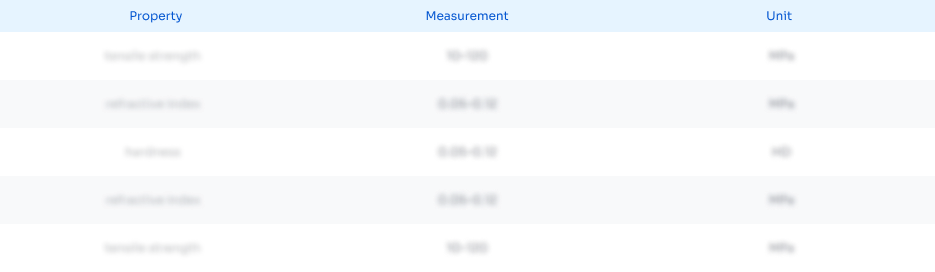
Abstract
Description
Claims
Application Information
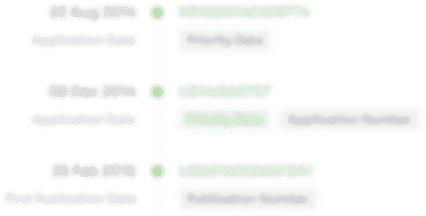
- R&D Engineer
- R&D Manager
- IP Professional
- Industry Leading Data Capabilities
- Powerful AI technology
- Patent DNA Extraction
Browse by: Latest US Patents, China's latest patents, Technical Efficacy Thesaurus, Application Domain, Technology Topic, Popular Technical Reports.
© 2024 PatSnap. All rights reserved.Legal|Privacy policy|Modern Slavery Act Transparency Statement|Sitemap|About US| Contact US: help@patsnap.com