Image retrieval method based on neighborhood rotation right angle mode
An image retrieval, right-angle technology, used in character and pattern recognition, special data processing applications, instruments, etc., can solve problems such as loss of partial image information, low recall and precision.
- Summary
- Abstract
- Description
- Claims
- Application Information
AI Technical Summary
Problems solved by technology
Method used
Image
Examples
specific Embodiment approach 1
[0035] Specific embodiment one: a kind of image retrieval method based on neighborhood rotation right angle pattern comprises the following steps:
[0036] Step 1: Separate the three-channel colors of the color image R, G, and B, select the haar wavelet base, and perform two-dimensional discrete wavelet transform on the three-channel colors respectively, and take the LL low-frequency subbands of the three-channel colors, namely cA_R, cA_G, and cA_B The low-frequency sub-band is used as the selected plane of the experiment; the R is a red component, G is a green component, B is a blue component, cA_R is a red component low-frequency sub-band, cA_G is a green component low-frequency sub-band, and cA_B is a blue component low-frequency sub-band bring;
[0037] Step 2: Based on the VLBP mode (Volume Local Binary Patterns volume local binary mode), the local pattern is extracted according to the plane selected in step 1;
[0038] Step 3: Using the neighborhood right-angle rotation m...
specific Embodiment approach 2
[0040] Specific embodiment two: the difference between this embodiment and specific embodiment one is: the specific process of extracting the local mode based on the VLBP mode in the step two according to the plane selected in the step one is:
[0041] Arrange the selected planes vertically, the order is that the first plane is the low frequency subband of the red component, the second plane is the low frequency subband of the blue component, and the third plane is the low frequency subband of the green component; A 3×3 pixel matrix formed by each pixel as the center, that is, a matrix formed by the center of the pixel and its surrounding eight neighboring pixels; the pixels around the plane expand outward, and its value is equal to its own pixel value;
[0042] The three planes are equivalent to a cube, that is, the 3×3 pixel matrix on the three planes is also equivalent to a cube, and the local mode of each 3×3 pixel matrix cube is extracted in turn, and the extracted local m...
specific Embodiment approach 3
[0044] Embodiment 3: The difference between this embodiment and Embodiment 1 or 2 is that in the step 3, the local pattern extracted in step 2 is calculated using the neighborhood right-angle rotation pattern of the local pattern. The specific process is as follows: :
[0045] Use the neighborhood rotation right-angle mode to calculate the extracted local mode to obtain a local three-valued mode, decompose each local three-valued mode into two local binary modes, and form a total of 10 local binary values encoded by 0,1 mode, the weighted operation is performed on the 10 local binary modes to obtain the values of 10 neighborhood rotation right-angle modes. The feature vector extraction process is as follows figure 1 shown.
[0046] Other steps and parameters are the same as those in Embodiment 1 or Embodiment 2.
PUM
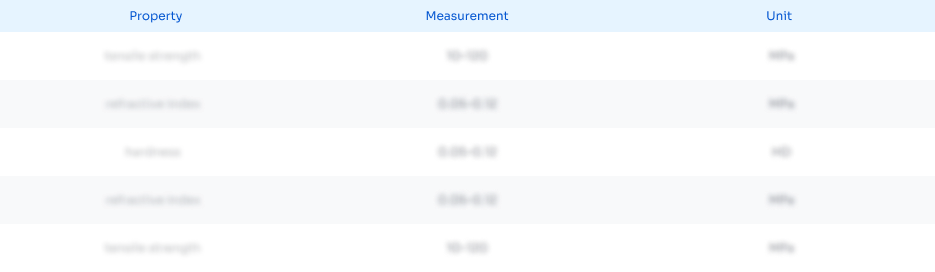
Abstract
Description
Claims
Application Information
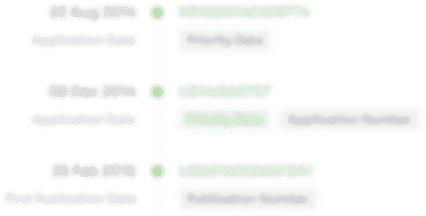
- R&D Engineer
- R&D Manager
- IP Professional
- Industry Leading Data Capabilities
- Powerful AI technology
- Patent DNA Extraction
Browse by: Latest US Patents, China's latest patents, Technical Efficacy Thesaurus, Application Domain, Technology Topic, Popular Technical Reports.
© 2024 PatSnap. All rights reserved.Legal|Privacy policy|Modern Slavery Act Transparency Statement|Sitemap|About US| Contact US: help@patsnap.com