Image target detection method based on natural language semantics
A target detection and natural language technology, applied in the field of image target detection based on natural language semantics, can solve problems such as not supporting end-to-end training, efficiency and accuracy need to be improved, and can not be combined with natural language target recognition to improve detection efficiency , the effect of improving the accuracy
- Summary
- Abstract
- Description
- Claims
- Application Information
AI Technical Summary
Problems solved by technology
Method used
Image
Examples
Embodiment Construction
[0018] In order to describe the present invention more specifically, the technical solutions of the present invention will be described in detail below in conjunction with the accompanying drawings and specific embodiments.
[0019] The present invention as figure 1 shown, including the following steps:
[0020] 1. Train the shared convolutional neural network and RPN network parts of the Faster-RCNN module on the ImageNet dataset.
[0021] 2. Use the image data with target natural semantic annotations given in the ReferIt dataset to train the LSTM model.
[0022] 3. For the trained model, given an image and a natural language phrase description of the target to be queried, the corresponding target is detected from the image.
[0023] Specifically, for the input image, first use the shared convolutional neural network to extract the feature map of the image. The convolutional neural network consists of a series of convolution, activation function activation and pooling opera...
PUM
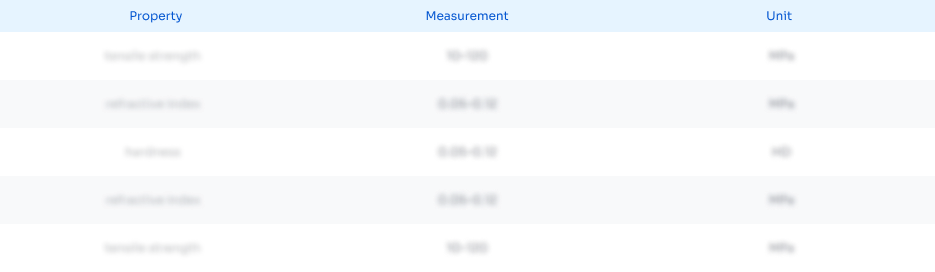
Abstract
Description
Claims
Application Information
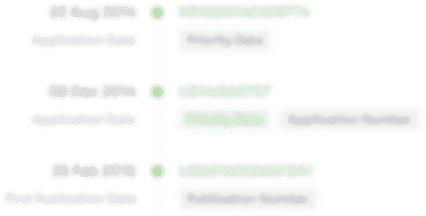
- R&D
- Intellectual Property
- Life Sciences
- Materials
- Tech Scout
- Unparalleled Data Quality
- Higher Quality Content
- 60% Fewer Hallucinations
Browse by: Latest US Patents, China's latest patents, Technical Efficacy Thesaurus, Application Domain, Technology Topic, Popular Technical Reports.
© 2025 PatSnap. All rights reserved.Legal|Privacy policy|Modern Slavery Act Transparency Statement|Sitemap|About US| Contact US: help@patsnap.com