Three-dimensional model classifying method based on feature matching
A three-dimensional model and classification method technology, applied in character and pattern recognition, special data processing applications, instruments, etc., can solve the problems of large workload of two-dimensional image classification, limited practical application scope, etc. Save time and effort, avoid the effect of multiple views
- Summary
- Abstract
- Description
- Claims
- Application Information
AI Technical Summary
Problems solved by technology
Method used
Image
Examples
Embodiment 2
[0055] The scheme in embodiment 1 is introduced in detail below in conjunction with specific calculation formulas and examples, see the following description for details:
[0056] 201: Define the multi-view color view set of each object in the training data as a multi-view training model library SD (SetDatabase), such as figure 2 As shown, a view is randomly selected from the multi-view color view set of each object to obtain an initial single-view view, and the single-view set of all objects is defined as a single-view training model library VD (View Database), such as image 3 shown;
[0057] 202: In the multi-view training model library and the single-view training model library, extract the CNN features of the initial view set of each object, and obtain the initial feature multi-view training vector set and category labels Initial Feature Single View Training Vector Set and category labels
[0058] Among them, the CNN feature, also known as the convolutional neur...
Embodiment 3
[0111] Combined with the specific experimental data, Figure 4 The scheme in embodiment 1 and 2 is carried out feasibility verification, see the following description for details:
[0112] The database used in this experiment is the database ETH published by Taiwan University of China [9] . This is a real-world multi-view model database, which contains a total of 80 objects in 8 categories, and each object has a total of 41 views. In this experiment, 24 objects of 3 types are selected as the training set, and the remaining objects are used as the set to be classified.
[0113] Several parameters are involved in this experiment: number of iterations, weight coefficient λ 1 ,λ 2 and the number of neighboring points k 1 , k 2 . In this experiment, the number of iterations is set to 10, and the weight coefficient λ 1 =0.9,λ 2 = 0.1 and the number of adjacent points k 1 = 2,k 2 =5. After classification, the category label of the 3D model is compared with its original ca...
PUM
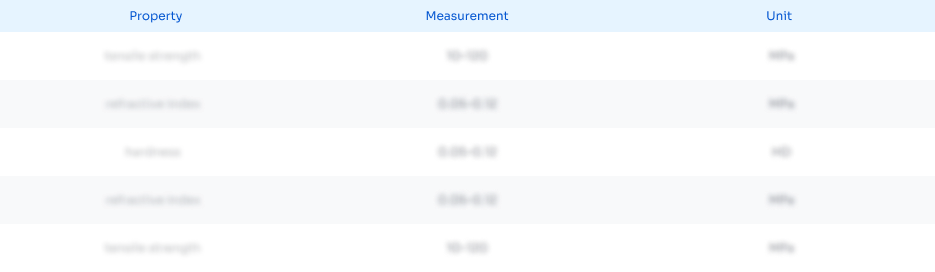
Abstract
Description
Claims
Application Information
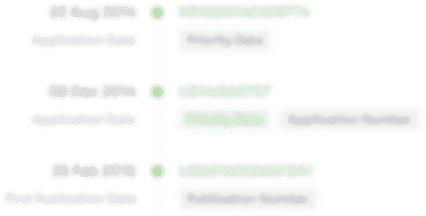
- Generate Ideas
- Intellectual Property
- Life Sciences
- Materials
- Tech Scout
- Unparalleled Data Quality
- Higher Quality Content
- 60% Fewer Hallucinations
Browse by: Latest US Patents, China's latest patents, Technical Efficacy Thesaurus, Application Domain, Technology Topic, Popular Technical Reports.
© 2025 PatSnap. All rights reserved.Legal|Privacy policy|Modern Slavery Act Transparency Statement|Sitemap|About US| Contact US: help@patsnap.com