Short-term wind power prediction method based on EWT-ESN
A wind power forecasting and wind power technology, applied in forecasting, instrumentation, data processing applications, etc., can solve the problems of forecasting point error and low forecasting accuracy
- Summary
- Abstract
- Description
- Claims
- Application Information
AI Technical Summary
Problems solved by technology
Method used
Image
Examples
Embodiment 1
[0072] Embodiment 1: as attached figure 1 , 2 , 3, and 4, the short-term wind power forecasting method based on EWT-ESN includes the following steps:
[0073] In the first step, the original wind power with strong nonlinearity and non-stationarity is decomposed using the EWT algorithm to obtain N modal components, where F 0 Denotes the empirical scale component, F 1 to F N Indicates the empirical wavelet component; that is, the calculation of the EWT decomposes the original signal f(t) into N+1 intrinsic mode functions f k (t), a f k (t) is defined as a group of amplitude modulation and frequency modulation signals, that is, AM-FM signals, and the expression formula is as follows:
[0074]
[0075] In the second step, combine the decomposition components F 0 to F N According to the characteristics of the time series, the ESN prediction model of each component is established, and the formula is as follows:
[0076]
[0077] Among them, η represents the number of p...
Embodiment 2
[0138] Embodiment 2: as attached Figure 5 , 6 , 7, 8 and table 1, shown in table 2, according to the actually measured wind power data between December 1, 2010 to the 27th of Canada's Alberta wind farm, the sampling interval of the original data set is 10min, in the present invention Take 30min as the sampling interval to obtain the experimental data set, take the first 80% of the data as training samples, and the remaining 20% of the data as testing samples.
[0139] The original data set is decomposed by EMD and EWT methods, and the decomposition results are shown in the attached Figure 5 And attached Figure 6 As shown, it can be seen that there is a big difference between the decomposition results of EWT and EMD. First, EWT decomposes to obtain 6 modal components, while EMD decomposes to obtain 8 modal components. number, which saves the calculation scale of combined forecasting; secondly, the IMF components obtained by EMD decomposition show the law of changing fro...
Embodiment 3
[0143] Embodiment 3: as attached Figure 9 , 10 , 11, 12, and Tables 3 and 4, according to the measured wind power data from September 23 to September 30 of the China Energy Conservation Wind Farm in Urumqi, Xinjiang, the sampling interval of the data set is 15 minutes. Get the data of the first 7 days as the training data set, and the data set of the 30th day (96 sample points) is used as the test data set to verify the prediction effect of the present invention.
[0144] According to attached Figure 9 And attached Figure 10 Shown, adopt EMD and EWT method to decompose the decomposition result figure that 8 days of raw data obtain, with embodiment 2, there is very big difference in the decomposition result of EWT and EMD, and EWT decomposition has obtained 5 modal components, and EMD decomposes to obtain 8 modal components; secondly, the amplitude of the component F0 decomposed by EWT accounts for a large proportion of the original original component x, and its change is...
PUM
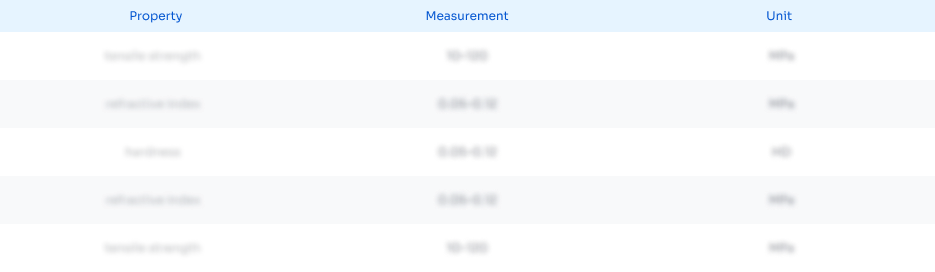
Abstract
Description
Claims
Application Information
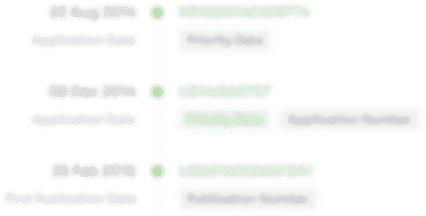
- R&D Engineer
- R&D Manager
- IP Professional
- Industry Leading Data Capabilities
- Powerful AI technology
- Patent DNA Extraction
Browse by: Latest US Patents, China's latest patents, Technical Efficacy Thesaurus, Application Domain, Technology Topic.
© 2024 PatSnap. All rights reserved.Legal|Privacy policy|Modern Slavery Act Transparency Statement|Sitemap