Industrial mechanical arm visual control method based on depth convolution neural network
A deep convolution and neural network technology, which is applied in the field of vision control of industrial robotic arms based on deep convolutional neural networks, can solve the problems of restricting the development of the robot industry, poor operability, and high complexity.
- Summary
- Abstract
- Description
- Claims
- Application Information
AI Technical Summary
Problems solved by technology
Method used
Image
Examples
Embodiment Construction
[0059] The present invention will be further described below in conjunction with specific examples.
[0060] Take the six-degree-of-freedom redundant industrial robot arm as an example, such as figure 1 As shown, the industrial manipulator vision control method based on the deep convolutional neural network of this embodiment specifically includes the following steps:
[0061] 1) Acquisition and preprocessing of target object visual information
[0062] Place the target object on the workbench, use the CCD camera to collect the color information pictures of the target object on the workbench in different postures, different positions, and different directions, and manually mark the ideal grasping pose points. The purpose is to fully obtain the visual information representation of the target object and mark the ideal grasping position, so as to fully represent the actual distribution of the target object in various situations. There can be many kinds of target objects, such a...
PUM
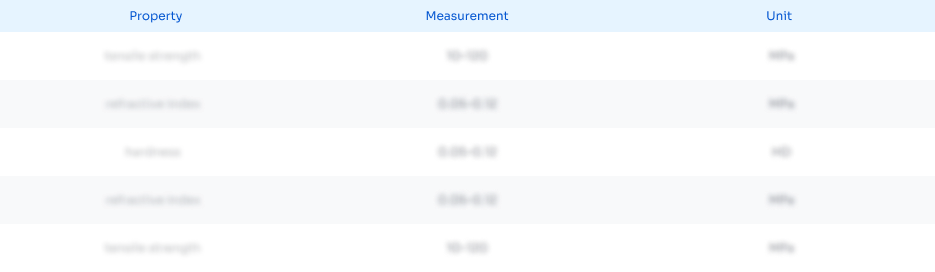
Abstract
Description
Claims
Application Information
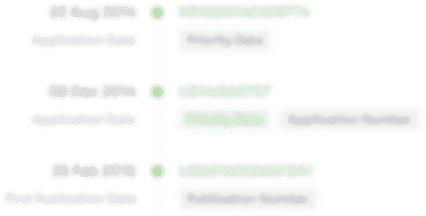
- R&D Engineer
- R&D Manager
- IP Professional
- Industry Leading Data Capabilities
- Powerful AI technology
- Patent DNA Extraction
Browse by: Latest US Patents, China's latest patents, Technical Efficacy Thesaurus, Application Domain, Technology Topic, Popular Technical Reports.
© 2024 PatSnap. All rights reserved.Legal|Privacy policy|Modern Slavery Act Transparency Statement|Sitemap|About US| Contact US: help@patsnap.com