Coarse-grained reconfigurable convolution neural network accelerator and system
A convolutional neural network, coarse-grained technology, applied in the field of high-efficiency hardware accelerator design, can solve the problems of low operation speed, large circuit scale, long development cycle, etc., to reduce reconfiguration overhead, reconfiguration time, and reconfiguration. Configure the effect of the speed boost
- Summary
- Abstract
- Description
- Claims
- Application Information
AI Technical Summary
Problems solved by technology
Method used
Image
Examples
Embodiment Construction
[0021] The specific implementation manners of the present invention will be further described in detail below in conjunction with the accompanying drawings and embodiments. The following examples are used to illustrate the present invention, but are not intended to limit the scope of the present invention.
[0022] figure 1 It shows a coarse-grained reconfigurable convolutional neural network accelerator, including multiple processing unit clusters, each processing unit cluster includes several basic computing units, and the several basic computing units are connected by a sub-addition unit, The sub-addition unit is as figure 1 In ADDB1-ADDB4, the sub-addition units of the plurality of processing unit clusters are respectively connected to a mother addition unit, and the mother addition unit is as figure 1 ADDB0 shown in, the sub-addition unit has the same structure as the mother addition unit; each sub-addition unit is used to generate the partial sum of adjacent basic addi...
PUM
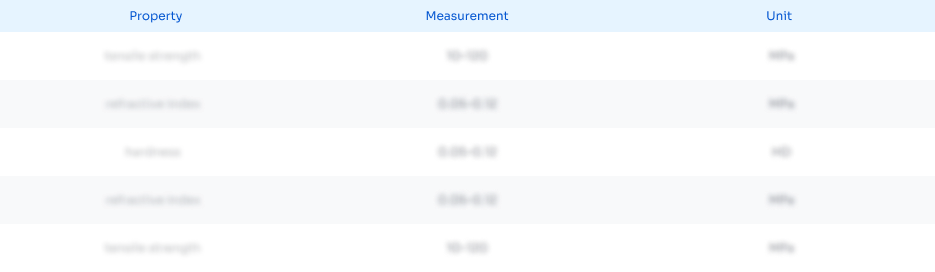
Abstract
Description
Claims
Application Information
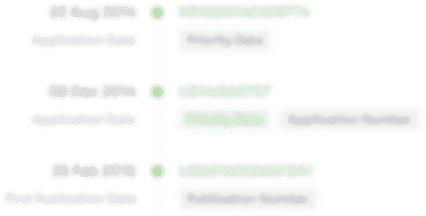
- R&D Engineer
- R&D Manager
- IP Professional
- Industry Leading Data Capabilities
- Powerful AI technology
- Patent DNA Extraction
Browse by: Latest US Patents, China's latest patents, Technical Efficacy Thesaurus, Application Domain, Technology Topic, Popular Technical Reports.
© 2024 PatSnap. All rights reserved.Legal|Privacy policy|Modern Slavery Act Transparency Statement|Sitemap|About US| Contact US: help@patsnap.com