State estimation method of nonlinear system
A nonlinear system and state estimation technology, applied in the field of state estimation of nonlinear systems, can solve the problems of limited accuracy of unscented Kalman filtering and reduced estimation performance, and achieve the effect of self-adaptive adjustment and improvement of estimation accuracy.
- Summary
- Abstract
- Description
- Claims
- Application Information
AI Technical Summary
Problems solved by technology
Method used
Image
Examples
Embodiment Construction
[0063] The implementation of the present application will be described in detail below with reference to the accompanying drawings and examples, so as to fully understand and implement the implementation process of how the present application uses technical means to solve technical problems and achieve technical effects.
[0064] State Space Models of Neural Networks
[0065] The structural models of neural networks can generally be divided into feedforward neural network models, feedback neural network models, and stochastic neural network models. At present, the feedforward neural network model is the most widely used in various industries. The model structure of its state space is as follows: figure 1 shown, where x 1 ,x 2 ,...x n The sample node used to represent the input, y 1 ,y 2 ,...y m Used to represent the output sample node, θ 1 ,θ 2 ,...θ q Represents the weight coefficient. The neural network model structure has three node layers, namely the input layer, hi...
PUM
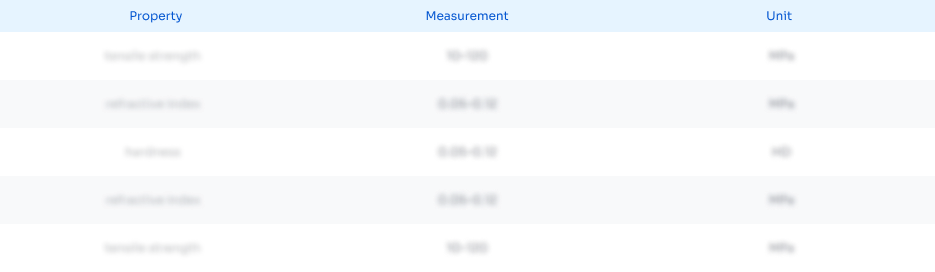
Abstract
Description
Claims
Application Information
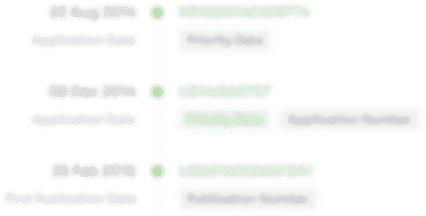
- R&D Engineer
- R&D Manager
- IP Professional
- Industry Leading Data Capabilities
- Powerful AI technology
- Patent DNA Extraction
Browse by: Latest US Patents, China's latest patents, Technical Efficacy Thesaurus, Application Domain, Technology Topic, Popular Technical Reports.
© 2024 PatSnap. All rights reserved.Legal|Privacy policy|Modern Slavery Act Transparency Statement|Sitemap|About US| Contact US: help@patsnap.com