De-convolutional neural network-based scene semantic segmentation method
A neural network and semantic segmentation technology, applied to instruments, character and pattern recognition, computer components, etc., can solve problems such as object classification errors, rough edges of segmented objects, etc., and achieve the effect of overcoming inherent defects and improving scene segmentation accuracy
- Summary
- Abstract
- Description
- Claims
- Application Information
AI Technical Summary
Problems solved by technology
Method used
Image
Examples
Embodiment Construction
[0024] Preferred embodiments of the present invention are described below with reference to the accompanying drawings. Those skilled in the art should understand that these embodiments are only used to explain the technical principles of the present invention, and are not intended to limit the protection scope of the present invention.
[0025] Such as figure 1 As shown, the scene semantic segmentation method based on the deconvolution neural network of an embodiment of the present invention comprises the following steps:
[0026] Step S1, using a fully convolutional neural network to extract low-resolution dense feature representations from scene images;
[0027] Step S2, using the locally sensitive deconvolutional neural network and the local affinity matrix of the picture to upsample and optimize the dense feature expression obtained in step S1 to obtain the score map of the picture, so as to achieve fine scene semantic segmentation.
[0028] Scene semantic segmentation ...
PUM
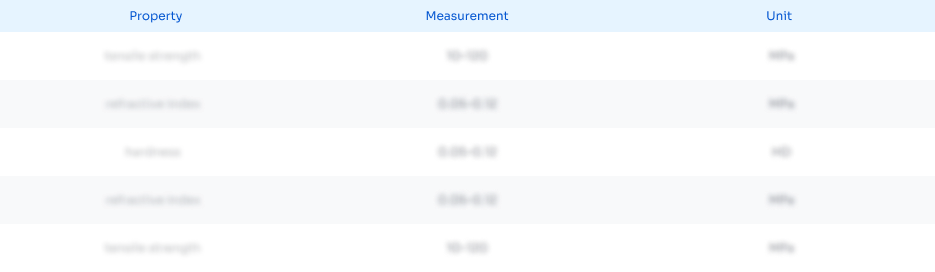
Abstract
Description
Claims
Application Information
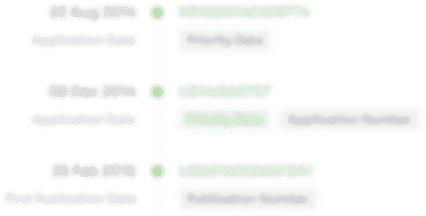
- Generate Ideas
- Intellectual Property
- Life Sciences
- Materials
- Tech Scout
- Unparalleled Data Quality
- Higher Quality Content
- 60% Fewer Hallucinations
Browse by: Latest US Patents, China's latest patents, Technical Efficacy Thesaurus, Application Domain, Technology Topic, Popular Technical Reports.
© 2025 PatSnap. All rights reserved.Legal|Privacy policy|Modern Slavery Act Transparency Statement|Sitemap|About US| Contact US: help@patsnap.com