Non-invasive load identification algorithm based on hybrid neural network and ensemble learning
A hybrid neural network and load recognition technology, applied in character and pattern recognition, biological neural network model, neural architecture, etc., can solve problems such as long training time, reduced recognition effect, unbalanced information content, etc., to achieve improved recognition effect, The effect of solving adverse effects and reducing variance
- Summary
- Abstract
- Description
- Claims
- Application Information
AI Technical Summary
Problems solved by technology
Method used
Image
Examples
Embodiment Construction
[0039] The present invention will be further described below in conjunction with the accompanying drawings and specific embodiments.
[0040] figure 1 It is a schematic diagram of the present invention. refer to figure 1 As shown, the user data that needs to be identified is first processed, and the data source is the data of house_3 in the REDD dataset. After the processing is completed, time-domain data such as voltage, current, and power are obtained. Then, the data is processed by discrete time-domain Fourier transform of non-periodic discrete signals to obtain the 3rd, 5th, and 7th harmonic characteristics of voltage and current, which are frequency domain data. Next, normalize the data, and the normalization method is linear function normalization. The processed data is input into a hybrid neural network composed of a recurrent neural network (RNN) and an artificial neural network (ANN), and the trained neural network will output the switching status of each electric...
PUM
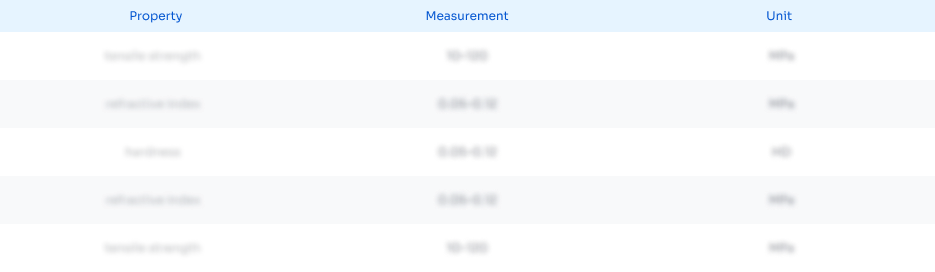
Abstract
Description
Claims
Application Information
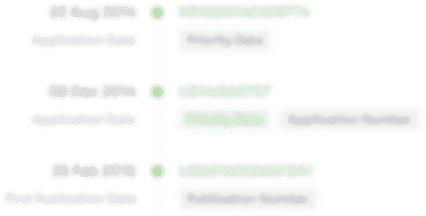
- R&D Engineer
- R&D Manager
- IP Professional
- Industry Leading Data Capabilities
- Powerful AI technology
- Patent DNA Extraction
Browse by: Latest US Patents, China's latest patents, Technical Efficacy Thesaurus, Application Domain, Technology Topic, Popular Technical Reports.
© 2024 PatSnap. All rights reserved.Legal|Privacy policy|Modern Slavery Act Transparency Statement|Sitemap|About US| Contact US: help@patsnap.com