Gray prediction and support vector machine-based classification type electric vehicle demand temporal-spatial distribution dynamic prediction method
A support vector machine and electric vehicle technology, applied in the field of electric power system, can solve the problems of blank space-time distribution dynamic prediction of electric vehicle demand by type and single consideration factors, etc.
- Summary
- Abstract
- Description
- Claims
- Application Information
AI Technical Summary
Problems solved by technology
Method used
Image
Examples
Embodiment Construction
[0082] One, the present invention mainly comprises the following steps:
[0083] The first step is to establish a model for predicting the number of classified vehicles. In this paper, through the analysis of the data characteristics of different types of vehicles such as regional buses, taxis, special vehicles, official vehicles and private passenger vehicles, the gray prediction method is selected for prediction; the research on the traditional gray prediction method aims at its limitations and different types. The characteristics of the vehicle data are improved to obtain an improved gray prediction model with high accuracy, and a forecast model for the number of classified vehicles is constructed; the development trend of different types of vehicles in the area obtained from the investigation is used to predict the number of different types of vehicles in the area through the forecast model of the number of classified vehicles general development trend in the future.
[0...
PUM
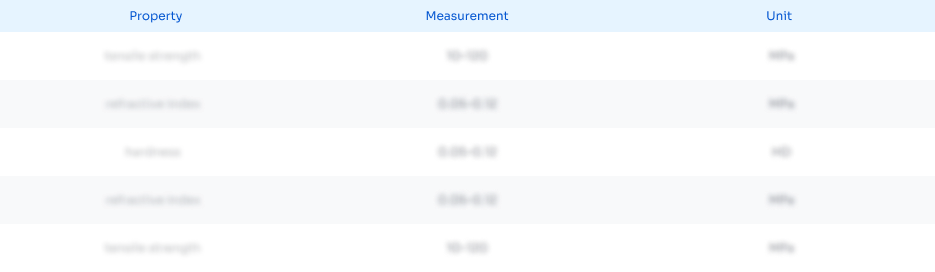
Abstract
Description
Claims
Application Information
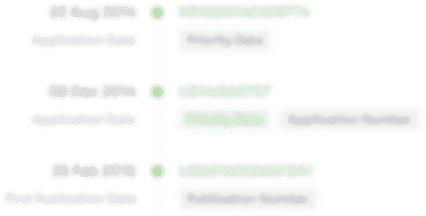
- R&D Engineer
- R&D Manager
- IP Professional
- Industry Leading Data Capabilities
- Powerful AI technology
- Patent DNA Extraction
Browse by: Latest US Patents, China's latest patents, Technical Efficacy Thesaurus, Application Domain, Technology Topic.
© 2024 PatSnap. All rights reserved.Legal|Privacy policy|Modern Slavery Act Transparency Statement|Sitemap