Multi-threshold image segmentation method based on crossover mutation artificial fish swarm algorithm
An artificial fish swarm algorithm and image segmentation technology, applied in the field of image processing, can solve problems such as weak exploration ability, weak development ability, loss of image information, etc., and achieve the effect of improving exploration ability, improving development ability, and enhancing diversity
- Summary
- Abstract
- Description
- Claims
- Application Information
AI Technical Summary
Problems solved by technology
Method used
Image
Examples
Embodiment Construction
[0039] The present invention will be described in further detail below in conjunction with the accompanying drawings.
[0040] The present invention improves the optimization ability of the original artificial fish swarm algorithm, introduces cross behavior and mutation behavior, and selects a suitable gray value as the segmentation threshold, so that each individual artificial fish in the group represents a group of possible solutions of the segmentation threshold , through a certain number of iterations, all possible solutions are updated based on the cross mutation artificial fish swarm algorithm. During each iteration, each artificial fish updates its own information by selecting different behavior patterns. When the maximum number of iterations is reached, it outputs the optimal threshold solution corresponding to the maximum value of the searched fitness function. Finally, according to the optimal threshold solution Split the image.
[0041] refer to figure 1 , the imp...
PUM
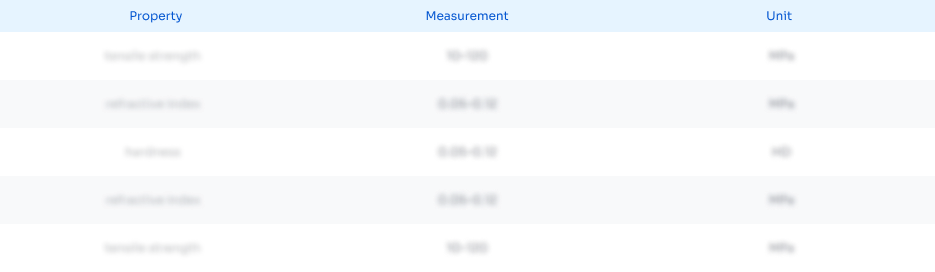
Abstract
Description
Claims
Application Information
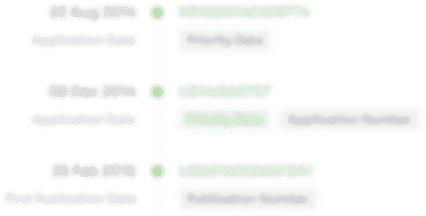
- R&D
- Intellectual Property
- Life Sciences
- Materials
- Tech Scout
- Unparalleled Data Quality
- Higher Quality Content
- 60% Fewer Hallucinations
Browse by: Latest US Patents, China's latest patents, Technical Efficacy Thesaurus, Application Domain, Technology Topic, Popular Technical Reports.
© 2025 PatSnap. All rights reserved.Legal|Privacy policy|Modern Slavery Act Transparency Statement|Sitemap|About US| Contact US: help@patsnap.com