Characteristic vector group's best selected spectrum clustering method based on density self-adaptation
An eigenvector and self-adaptive technology, applied in the field of spectral clustering algorithm, can solve problems such as unsatisfactory multi-scale data sets, difficulty in selecting eigenvector groups, and manually determining the number of clusters.
- Summary
- Abstract
- Description
- Claims
- Application Information
AI Technical Summary
Problems solved by technology
Method used
Image
Examples
Embodiment Construction
[0070] The present invention will be further described below in conjunction with the accompanying drawings.
[0071] refer to Figure 1 to Figure 5 , a density adaptive eigenvector group optimal selection spectral clustering method, including the following steps:
[0072] 1) Data preprocessing:
[0073] 1.1) Initialize the settings, determine the iteration range of the number of adjacent points and the initial number of adjacent points, set the initial Fitness function value to Fitness_g=0, and divide the interval number block;
[0074] 1.2) Analyzing the actual data set, we can see that some dimensions of some data sets are much larger than other dimensions of the data set, and the difference between the values of these dimensions is large, which may lead to the weakening of the importance of other dimensions even ignored. Without obtaining the weight information of each dimension of the data set, the minimum-maximum method is used to normalize the data so that it falls ...
PUM
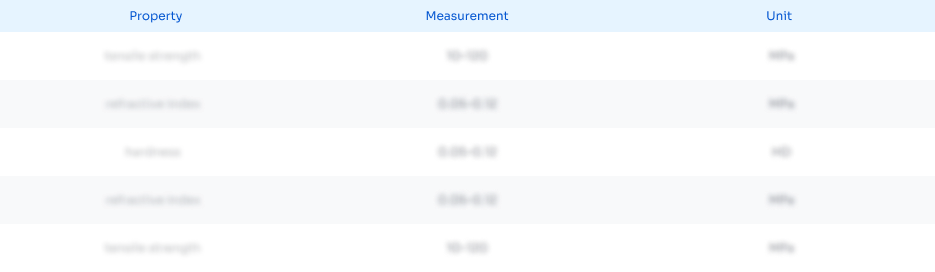
Abstract
Description
Claims
Application Information
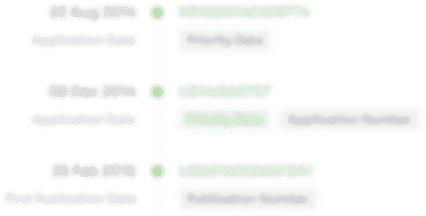
- R&D Engineer
- R&D Manager
- IP Professional
- Industry Leading Data Capabilities
- Powerful AI technology
- Patent DNA Extraction
Browse by: Latest US Patents, China's latest patents, Technical Efficacy Thesaurus, Application Domain, Technology Topic.
© 2024 PatSnap. All rights reserved.Legal|Privacy policy|Modern Slavery Act Transparency Statement|Sitemap