Depth convolution wavelet neural network expression identification method based on auxiliary task
A wavelet neural network and deep convolution technology, applied in the field of image processing, can solve the problems of small improvement in classification effect, failure to avoid feature selection, and inability to learn expression features, so as to improve generalization ability, improve accuracy, and improve The effect of influence
- Summary
- Abstract
- Description
- Claims
- Application Information
AI Technical Summary
Problems solved by technology
Method used
Image
Examples
Embodiment 1
[0043]Facial expression recognition is an indispensable part of machine learning research. It has very broad application value in today's society where human-computer interaction is becoming more and more popular. In human-machine interfaces such as mobile terminals and personal computers, the recognition of human facial expressions Real-time automatic recognition; in some occasions, facial expressions are retrieved from videos for tracking and recognition. The breakthrough of facial expression recognition method also has great reference significance for the field of intelligent computing and brain-inspired research.
[0044] In the existing facial expression recognition technology, it is mainly the method of feature selection and then classification, but in the feature selection step, the existing feature selection operator cannot efficiently learn the expression features, so that the subsequent classification cannot be obtained. ideal result. In addition, the method of usin...
Embodiment 2
[0081] The deep convolution wavelet neural network expression recognition method based on auxiliary task is the same as embodiment 1, and the described set-up facial expression image set and expression sensitive area image set of step (2) are carried out according to the following steps:
[0082] 2.1 The facial expression image set is obtained as follows:
[0083] Randomly select the original image with label of suitable quantity from JAFFE expression image storehouse, the image in the JAFFE expression image storehouse that the present invention adopts, as attached figure 1 As shown, there are 213 images in the image library, including seven types of expressions, namely: angry, sad, happy, calm, disgusted, surprised, and feared. The original image size is 256*256, see figure 1 , figure 1Listed some images of four people with different expressions, the first row shows angry expressions, the second row shows disgusted expressions, the third row shows frightened expressions, an...
Embodiment 3
[0088] The deep convolution wavelet neural network expression recognition method based on auxiliary tasks is the same as embodiment 1-2, and the wavelet pooling layer described in step (9) obtains low-frequency subbands and high-frequency subbands, referring to Fig. 3 (a), Fig. 3 In (a), the traditional downsampling pooling layer is transformed into a wavelet pooling layer. On the one hand, it avoids the information loss caused by simple downsampling, and on the other hand, it retains high-frequency information and enhances the local information of expression features. Follow the steps below:
[0089] 9.1 Perform a layer of downsampling wavelet decomposition on the feature map obtained by the previous layer of convolutional layer. The selected wavelet basis function is the Haar function. Each feature map is decomposed by a layer of downsampling wavelet to obtain a low frequency subband and a horizontal direction The high-frequency sub-band of , a vertical high-frequency sub-ba...
PUM
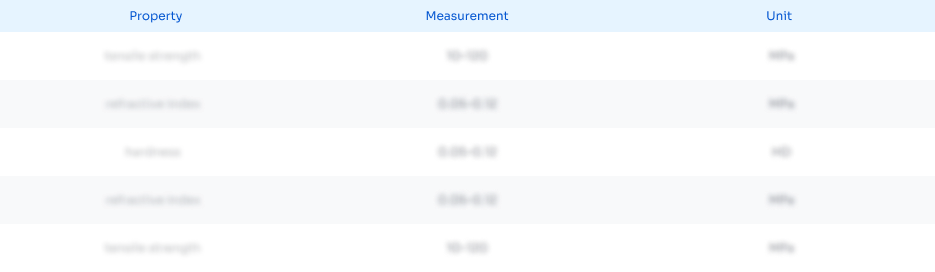
Abstract
Description
Claims
Application Information
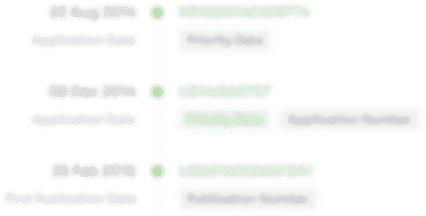
- R&D
- Intellectual Property
- Life Sciences
- Materials
- Tech Scout
- Unparalleled Data Quality
- Higher Quality Content
- 60% Fewer Hallucinations
Browse by: Latest US Patents, China's latest patents, Technical Efficacy Thesaurus, Application Domain, Technology Topic, Popular Technical Reports.
© 2025 PatSnap. All rights reserved.Legal|Privacy policy|Modern Slavery Act Transparency Statement|Sitemap|About US| Contact US: help@patsnap.com