SAR image semantic segmentation method for fast ridgelet deconvolution structure learning model
A learning model and deconvolution technology, applied in the field of image processing, can solve the problems of ignoring image structure information, limiting the scope of application, and taking a long time to achieve the effect of reducing training time, improving training efficiency, and reducing complexity
- Summary
- Abstract
- Description
- Claims
- Application Information
AI Technical Summary
Problems solved by technology
Method used
Image
Examples
Embodiment Construction
[0050] The present invention will be further described below in conjunction with the accompanying drawings.
[0051] Reference attached figure 1 , the realization steps of the present invention are as follows.
[0052] Step 1, according to the sketch model of the synthetic aperture radar SAR image, extract the sketch map of the synthetic aperture radar SAR image.
[0053] enter figure 2 For the SAR image shown, the sketch of the SAR image is obtained according to the sketch model of the SAR image, such as image 3 shown.
[0054] For the sketch model of the SAR image, refer to the article "Local maximal homogenous regionsearch for SAR speckle reduction with sketch-based geometrical kernel function" published by Jie-Wu et al. in IEEE Transactionson Geoscience and Remote Sensing magazine in 2014,
[0055] According to the sketch model of the SAR image, the sketch map of the SAR image is obtained, and the steps are as follows:
[0056] (1.1) Construct edge and line template...
PUM
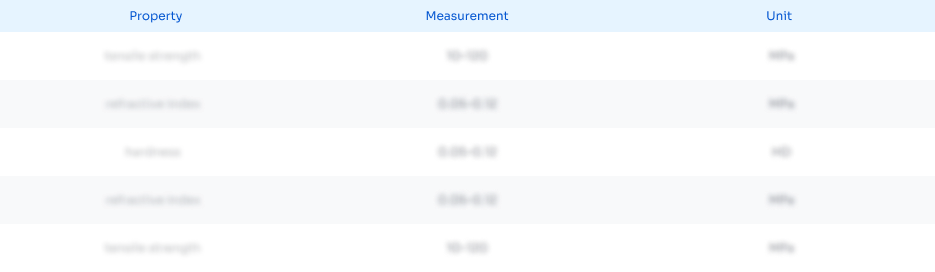
Abstract
Description
Claims
Application Information
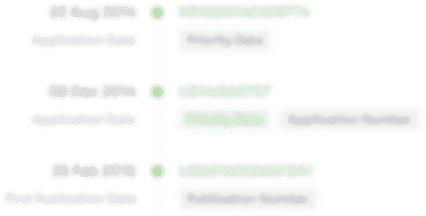
- R&D Engineer
- R&D Manager
- IP Professional
- Industry Leading Data Capabilities
- Powerful AI technology
- Patent DNA Extraction
Browse by: Latest US Patents, China's latest patents, Technical Efficacy Thesaurus, Application Domain, Technology Topic, Popular Technical Reports.
© 2024 PatSnap. All rights reserved.Legal|Privacy policy|Modern Slavery Act Transparency Statement|Sitemap|About US| Contact US: help@patsnap.com