Multi-mark classification method, device, medium and computing device
A classification method and multi-label technology, applied in computing, computer components, instruments, etc., can solve problems such as inaccurate classification results, and achieve the effect of enriching data and semantics
- Summary
- Abstract
- Description
- Claims
- Application Information
AI Technical Summary
Problems solved by technology
Method used
Image
Examples
Embodiment 1
[0029] refer to figure 1 , which is a schematic flow chart of the multi-label classification method provided in Embodiment 1 of the present application, the method includes the following steps:
[0030] Step 101: For each label in the label set, determine the original positive example set and the original negative example set of the label; wherein, for each sample, if the sample has the label, the sample belongs to the original positive example of the label set, otherwise, the sample belongs to the labeled original set of negative examples.
[0031] Step 102: Carry out class alignment on the original positive example set and the original negative example set of each mark respectively, and obtain the positive example set after the class alignment of each mark and the negative example set after the class alignment; wherein, the class-aligned set of each mark The number of samples in the positive example set is equal, and the number of samples in the negative example set after t...
Embodiment 2
[0084] As shown in Table 1, it is a multi-label data set. There are a total of 6 samples in this table, and are x 1 ,x 2 ,...,x 6 , the mark set is {l 1 , l 2}.
[0085] Table 1 Samples and the marks they have
[0086]
[0087] Step 1: Class Alignment:
[0088] It can be seen from the statistical table 1 that l 1 The original set of positive examples is Its original set of negative examples is l 2 The original set of positive examples is Its original set of negative examples is
[0089] Obviously, (i.e. l 1 The number of positive samples of is 2), therefore In order to achieve the and For class alignment, you only need to add a positive example to Just go there. optional l 1 2 positive examples in x 2 and x 3 to generate positive examples (x 2 +x 3 ) / 2. Similarly, in this example l 2There are fewer negative examples, and class alignment is also required. You can choose l 2 2 negative examples of x 3 and x 4 to generate negative examp...
Embodiment 3
[0113] Based on the same inventive concept, the embodiment of the present application also provides a multi-label classification device, such as figure 2 Shown is a schematic structural diagram of the device, including:
[0114] The positive and negative example set determination module 201 is used to determine the original positive example set and the original negative example set of the label for each label in the label set; wherein, for each sample, if the sample has the label, the The sample belongs to the original positive example set of the label, otherwise, the sample belongs to the original negative example set of the label;
[0115] The class alignment module 202 is used to perform class alignment on the original positive example set and the original negative example set of each mark respectively, and obtain the positive example set after the class alignment of each mark and the negative example set after the class alignment; wherein, each marked The number of sampl...
PUM
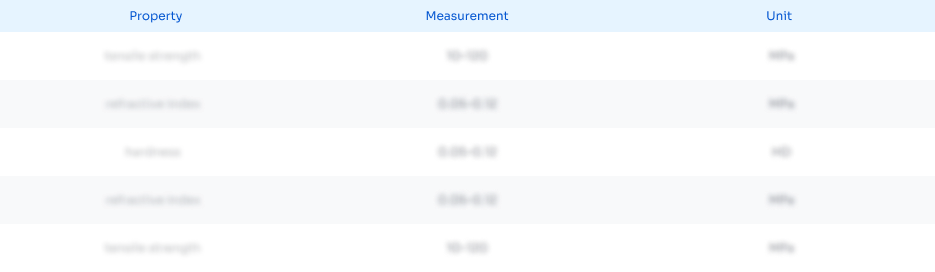
Abstract
Description
Claims
Application Information
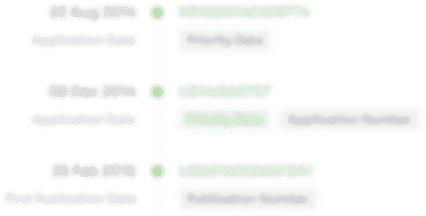
- R&D Engineer
- R&D Manager
- IP Professional
- Industry Leading Data Capabilities
- Powerful AI technology
- Patent DNA Extraction
Browse by: Latest US Patents, China's latest patents, Technical Efficacy Thesaurus, Application Domain, Technology Topic, Popular Technical Reports.
© 2024 PatSnap. All rights reserved.Legal|Privacy policy|Modern Slavery Act Transparency Statement|Sitemap|About US| Contact US: help@patsnap.com