Scene semantic segmentation method based on full convolution and long and short term memory units
A long-term and short-term memory and semantic segmentation technology, applied in the field of image semantic segmentation and deep learning, can solve the problems of over-segmentation of objects and low accuracy of scene image segmentation, and achieve the effect of solving low accuracy and improving accuracy.
- Summary
- Abstract
- Description
- Claims
- Application Information
AI Technical Summary
Problems solved by technology
Method used
Image
Examples
Embodiment Construction
[0027] In order to make the objectives, technical solutions, and advantages of the present invention clearer, the present invention will be further described in detail below in conjunction with specific implementation and drawings.
[0028] Such as figure 1 As shown, the specific steps of the scene semantic segmentation method based on full convolution and long short-term memory unit in this embodiment are as follows:
[0029] S1: Build a deep neural network based on full convolution, multi-scale fusion and long and short-term memory units.
[0030] Such as figure 2 As shown, the basic structure of the front-end convolutional neural network module is modified from VGG-16. The main components of VGG-16 are 5 group convolutional layers, 3 fully connected layers, and 1 Softmax layer. In this embodiment Use the front-end network to use the convolutional layer of the first 5 groups of VGG-16, and remove the pooling layer of the 4th and 5th group and the last 3 fully connected layers. Am...
PUM
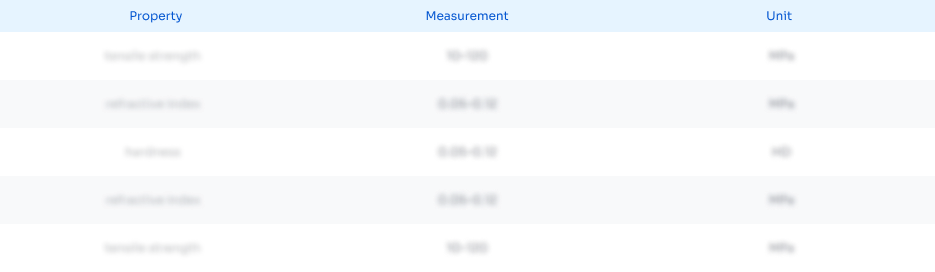
Abstract
Description
Claims
Application Information
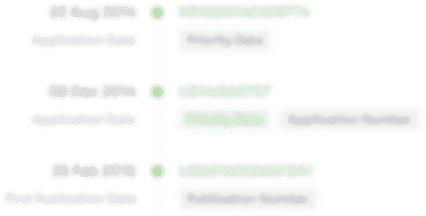
- R&D
- Intellectual Property
- Life Sciences
- Materials
- Tech Scout
- Unparalleled Data Quality
- Higher Quality Content
- 60% Fewer Hallucinations
Browse by: Latest US Patents, China's latest patents, Technical Efficacy Thesaurus, Application Domain, Technology Topic, Popular Technical Reports.
© 2025 PatSnap. All rights reserved.Legal|Privacy policy|Modern Slavery Act Transparency Statement|Sitemap|About US| Contact US: help@patsnap.com