Electrocardiogram signal classifying method based on one-dimensional convolutional neural network
A convolutional neural network and electrocardiographic signal technology, applied in medical science, sensors, diagnostic recording/measurement, etc., can solve the problems of noise sensitivity, complex data preprocessing, etc., and achieve the effect of avoiding noise sensitivity and solving precise positioning
- Summary
- Abstract
- Description
- Claims
- Application Information
AI Technical Summary
Problems solved by technology
Method used
Image
Examples
Embodiment Construction
[0050] In order to make the object, technical solution and advantages of the present invention clearer, the present invention will be described in detail below in conjunction with the accompanying drawings and specific embodiments.
[0051] The invention provides a method for classifying ECG signals based on a one-dimensional convolutional neural network. The ECG signal data used comes from the MIT-BIH standard arrhythmia database. The database contains 48 dual-channel dynamic ECG records, the first 23 Recordings were extracted from routine outpatient practice, while the remaining 25 recordings were selected for the presence of uncommon complex ventricular, junctional, supraventricular arrhythmia signals, each recording was 30 minutes long, and the sampling frequency was 360HZ. The present invention selects 44 recorded ECG signals of lead II from the database to train and verify the feasibility of the invention. Specifically include:
[0052] Step 1: Use the wavelet fusion m...
PUM
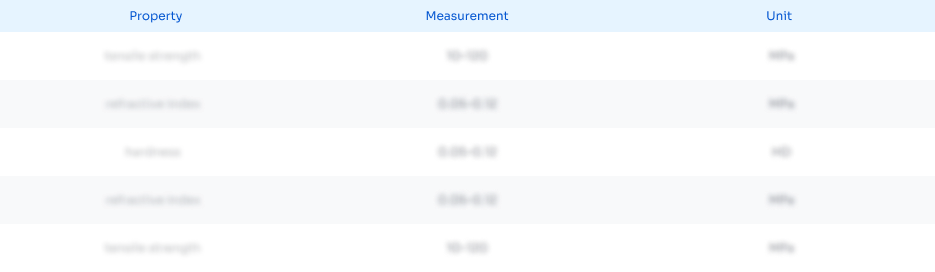
Abstract
Description
Claims
Application Information
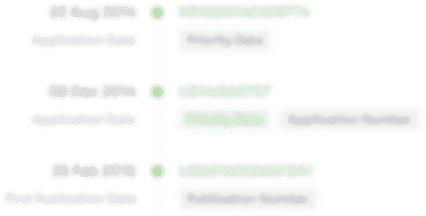
- R&D Engineer
- R&D Manager
- IP Professional
- Industry Leading Data Capabilities
- Powerful AI technology
- Patent DNA Extraction
Browse by: Latest US Patents, China's latest patents, Technical Efficacy Thesaurus, Application Domain, Technology Topic, Popular Technical Reports.
© 2024 PatSnap. All rights reserved.Legal|Privacy policy|Modern Slavery Act Transparency Statement|Sitemap|About US| Contact US: help@patsnap.com