Nonlinear industrial process fault detection method based on kernel principal component analysis
A nuclear principal component analysis and industrial process technology, applied in electrical testing/monitoring, etc., can solve problems such as inability to guarantee nuclear components, obey Gaussian distribution, and failure to effectively reflect fault information
- Summary
- Abstract
- Description
- Claims
- Application Information
AI Technical Summary
Problems solved by technology
Method used
Image
Examples
Embodiment 1
[0111] Embodiment 1: A nonlinear numerical system is taken as an example for illustration. Simulate a system with six monitored variables [x 1 ,x 2 ,x 3 ,x 4 ,x 5 ,x 6 ] nonlinear numerical system, its mathematical description is as follows:
[0112] x 1 = r 1 +e 1 (31)
[0113] x 2 = r 2 +e 2
[0114] x 3 = 2r 1 +3r 2 +e 3
[0115] x 4 =5r 1 -2r 2 +e 4
[0116] x 5 = r 1 2 -3r 2 +e 5
[0117] x 6 =-r 1 3 +3r 2 2 +e 6
[0118] In formula (31), e 1 ,e 2 ,e 3 ,e 4 ,e 5 ,e 6 ∈N(0,0.01) represents six independent Gaussian noise variables, r 1 ,r 2 ∈[0,2] is an independent uniformly distributed random variable, the output of the system [x 1 ,x 2 ,x 3 ,x 4 ,x 5 ,x 6 ] as a process monitoring variable. 400 samples are simulated under normal operating conditions shown in formula (31) as training data for modeling. In order to generate fault data, the variable x is changed at the 201st moment of the simulation 6 Add a sine wave with ...
Embodiment 2
[0201] Implementation example 2: The Tennessee-Eastman (TE) process is an experimental platform established by Downs and Vogel of Eastman Chemical Company in the United States based on an actual chemical process. It is now widely used to verify the optimality of control algorithms and process monitoring methods. inferior. The TE process is mainly composed of five units, including a reactor, a product condenser, a gas-liquid separator, a circulating compressor and a stripping tower. The structure diagram is as follows image 3 shown. The TE process has a total of 53 variables, including 22 continuous measurement variables, 19 component variables and 12 operational variables. This embodiment uses 33 variables in the TE process, as shown in Table 2. The 21 faults used in this embodiment are shown in Table 3, and are used to verify the monitoring performance of each method.
[0202] 33 variables of the TE process that the present embodiment of table 2 adopts
[0203] v...
PUM
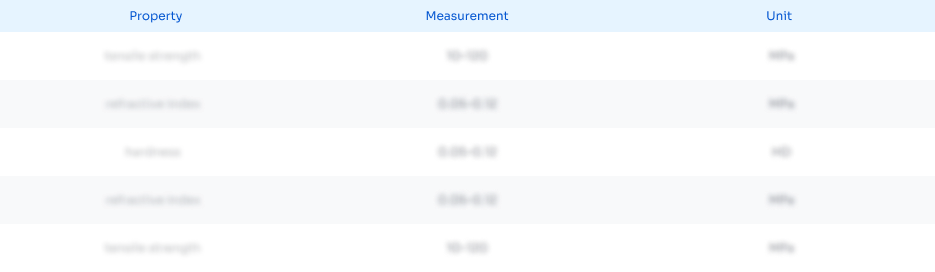
Abstract
Description
Claims
Application Information
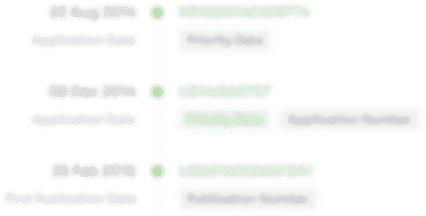
- R&D Engineer
- R&D Manager
- IP Professional
- Industry Leading Data Capabilities
- Powerful AI technology
- Patent DNA Extraction
Browse by: Latest US Patents, China's latest patents, Technical Efficacy Thesaurus, Application Domain, Technology Topic.
© 2024 PatSnap. All rights reserved.Legal|Privacy policy|Modern Slavery Act Transparency Statement|Sitemap