A Fault Detection Method for Nonlinear Industrial Processes Based on Bayesian Kernel Slow Eigen Analysis
A fault detection and industrial process technology, applied in electrical testing/monitoring, testing/monitoring control systems, instruments, etc., can solve problems such as inability to accurately extract non-linear characteristic information of data
- Summary
- Abstract
- Description
- Claims
- Application Information
AI Technical Summary
Problems solved by technology
Method used
Image
Examples
Embodiment 1
[0089] Embodiment 1: A nonlinear numerical system is taken as an example for illustration. simulates a system with three monitored variables x 1 ,x 2 ,x 3 The nonlinear numerical system of , its mathematical description is as follows:
[0090]
[0091] where e 1 ,e 2 ,e 3∈N(0,0.01) represents three independent noise variables, t∈[0,2] is a uniformly distributed random variable, and the output of the system [x 1 ,x 2 ,x 3 ] as a process monitoring variable. Under the normal operating conditions shown in formula (25), 300 samples are simulated as training data for modeling. In order to generate fault data, the variable x 1 Add ramp fault F1 and make fault F1 last until the end of the simulation at the 300th moment.
[0092] The fault detection method of the above-mentioned nonlinear numerical system comprises the following steps:
[0093] (1) Collect the normal operating condition data of the nonlinear numerical system as the training data X o , calculate the tra...
Embodiment 2
[0163] Embodiment two: Take the continuous stirred reactor (CSTR) system as an example, see Figure 4 , in the CSTR system, material A enters the reactor, a first-order irreversible chemical reaction occurs, material B is generated, heat is released, and the reactor is cooled by the jacketed coolant outside. In order to ensure the normal operation of the process, a cascade control system is adopted Control the liquid level and temperature of the reactor.
[0164] According to the process mechanism, the dynamic mechanism model of the CSTR system is established as follows:
[0165]
[0166]
[0167]
[0168]
[0169] In the formula, A is the cross-sectional area of the reactor, c A is the concentration of material A in the reactor, c AF is the concentration of material A in the feed, C p is the specific heat of the reactants, C pC is the coolant specific heat, E is the activation energy, h is the reactor liquid level, k 0 is the response factor, Q F Feed flow...
PUM
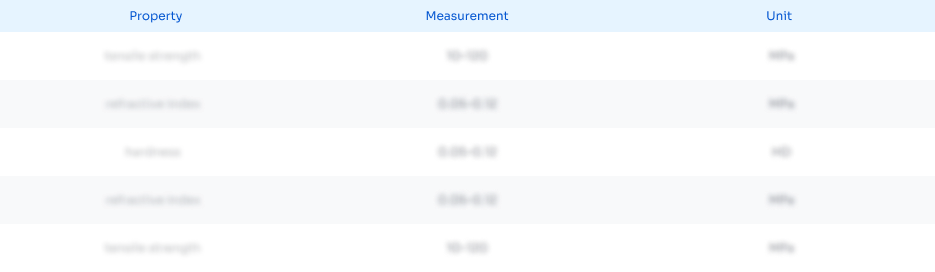
Abstract
Description
Claims
Application Information
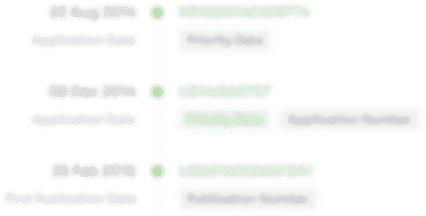
- Generate Ideas
- Intellectual Property
- Life Sciences
- Materials
- Tech Scout
- Unparalleled Data Quality
- Higher Quality Content
- 60% Fewer Hallucinations
Browse by: Latest US Patents, China's latest patents, Technical Efficacy Thesaurus, Application Domain, Technology Topic, Popular Technical Reports.
© 2025 PatSnap. All rights reserved.Legal|Privacy policy|Modern Slavery Act Transparency Statement|Sitemap|About US| Contact US: help@patsnap.com