Deep-learning-based multi-target pedestrian detection and tracking method
A technology of pedestrian detection and deep learning, applied in the field of computer vision, can solve the problems of pedestrians not being detected, re-identified, and obstacles blocked without processing, and achieve the effect of improving the tracking effect and the matching effect.
- Summary
- Abstract
- Description
- Claims
- Application Information
AI Technical Summary
Problems solved by technology
Method used
Image
Examples
Embodiment Construction
[0047] Specific embodiments of the present invention will be described in detail below with reference to the accompanying drawings.
[0048] Such as figure 1 As shown, a kind of deep learning-based multi-target pedestrian detection and tracking method of the present invention comprises the following steps:
[0049] Step 1: Perform multi-target pedestrian detection and joint point extraction for the input video frame sequence, and save the obtained position information and joint point information as the input for the next stage. Specifically, it is implemented through the following steps:
[0050] 1.1 First, in the preparatory stage of this method, a ResNet-based pedestrian detection convolutional neural network is trained to extract the visual features of the video frame. The convolutional network uses such as figure 2 The repetition of the unit, x is the input of the neural network signal, x is added to x itself after passing through the two-layer neural network, and then ...
PUM
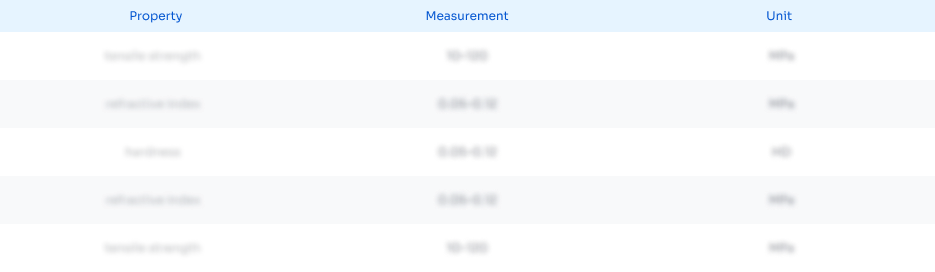
Abstract
Description
Claims
Application Information
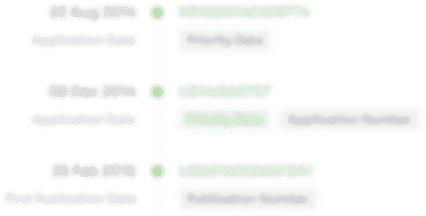
- R&D
- Intellectual Property
- Life Sciences
- Materials
- Tech Scout
- Unparalleled Data Quality
- Higher Quality Content
- 60% Fewer Hallucinations
Browse by: Latest US Patents, China's latest patents, Technical Efficacy Thesaurus, Application Domain, Technology Topic, Popular Technical Reports.
© 2025 PatSnap. All rights reserved.Legal|Privacy policy|Modern Slavery Act Transparency Statement|Sitemap|About US| Contact US: help@patsnap.com