Micro-operating system target detection method
An operating system and target detection technology, applied in the fields of computer vision and deep learning, can solve the problems of falling, the inability of different pose targets to be effectively recognized and recognized in real time, etc., to simplify the detection process, high-performance classification capabilities, ensure accuracy and real-time performance. sexual effect
- Summary
- Abstract
- Description
- Claims
- Application Information
AI Technical Summary
Problems solved by technology
Method used
Image
Examples
Embodiment 1
[0034] Use the deep residual convolutional neural network to obtain the feature map of the image to be recognized at the end of the manipulator, combine the target sample candidate frame obtained by the region proposal network, and perform target recognition through the region of interest pooling layer and the trained fully connected classification network. The target candidate frame of the image to be recognized at the end of the manipulator is obtained as the target recognition result of the micro-operating system.
Embodiment 2
[0036] For the image to be recognized of the vacuum gripper, the feature map is obtained by using the deep residual convolutional neural network, combined with the target sample candidate frame obtained by the region proposal network, through the region of interest pooling layer and the trained fully connected classification network, the target Recognition, the target candidate frame of the image to be recognized of the vacuum gripper is obtained as the target recognition result of the micro-operating system.
Embodiment 3
[0038] Use the deep residual convolutional neural network to obtain the feature map of the image to be identified in the column cavity, combine the target sample candidate frame obtained by the region proposal network, and perform target recognition through the region of interest pooling layer and the trained fully connected classification network. The target candidate frame of the to-be-recognized image of the column cavity is obtained as the target recognition result of the micro-operating system.
PUM
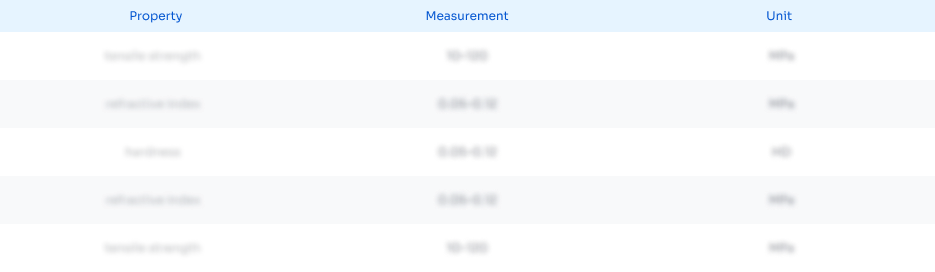
Abstract
Description
Claims
Application Information
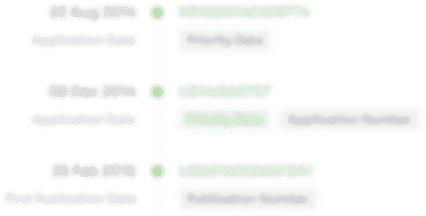
- R&D Engineer
- R&D Manager
- IP Professional
- Industry Leading Data Capabilities
- Powerful AI technology
- Patent DNA Extraction
Browse by: Latest US Patents, China's latest patents, Technical Efficacy Thesaurus, Application Domain, Technology Topic.
© 2024 PatSnap. All rights reserved.Legal|Privacy policy|Modern Slavery Act Transparency Statement|Sitemap