Speech recognition method based on neural network stacking autoencoder multi-feature fusion
A stacked autoencoder and multi-feature fusion technology, applied in neural learning methods, biological neural network models, speech recognition, etc. Recognition rate and calculation efficiency, speed up, and the effect of reducing feature dimension
- Summary
- Abstract
- Description
- Claims
- Application Information
AI Technical Summary
Problems solved by technology
Method used
Image
Examples
Embodiment Construction
[0038] Taking four types of excavation equipment (including hand-held electric picks, excavators, cutters, and hydraulic impact hammers) as examples, the Linear Prediction Cepstrum Coefficients (LPCC) and Mel frequency cepstral coefficients (Mel Frequency Cepstrum Coefficients, MFCC) these two feature extraction methods, the present invention is described further. The following description is only for demonstration and explanation, and does not limit the present invention in any form.
[0039] Model training:
[0040] Step 1. Carry out frame-based windowing on the collected sound data of the four types of excavation equipment during operation. The frame length is N, and the frame shift is Add the Hamming window to get the sound database;
[0041] Step 2, use the LPCC feature extraction algorithm to perform feature extraction on the sound source data of each frame, where the order of LPCC (i.e. the number of LPCC features) we record as R LPCC .
[0042] Step 3. Use the MFC...
PUM
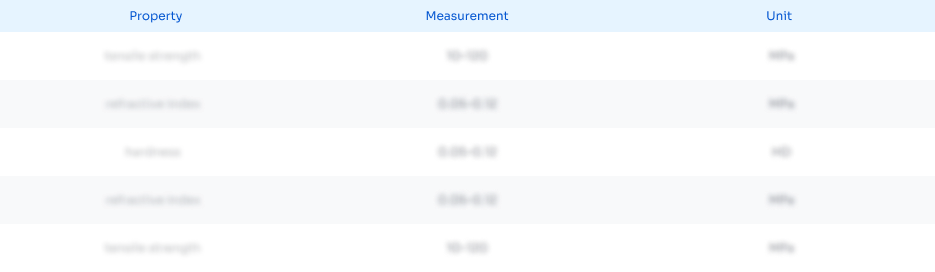
Abstract
Description
Claims
Application Information
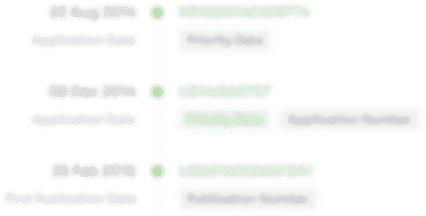
- R&D Engineer
- R&D Manager
- IP Professional
- Industry Leading Data Capabilities
- Powerful AI technology
- Patent DNA Extraction
Browse by: Latest US Patents, China's latest patents, Technical Efficacy Thesaurus, Application Domain, Technology Topic, Popular Technical Reports.
© 2024 PatSnap. All rights reserved.Legal|Privacy policy|Modern Slavery Act Transparency Statement|Sitemap|About US| Contact US: help@patsnap.com