A Model-Driven Adaptive Time-Frequency Transformation Method for Polynomial Phase Signals
A phase signal, time-frequency transformation technology, applied in the field of signal processing, can solve problems such as difficulty, high signal-to-noise ratio threshold, and insufficient capacity
- Summary
- Abstract
- Description
- Claims
- Application Information
AI Technical Summary
Problems solved by technology
Method used
Image
Examples
Embodiment Construction
[0066] The present invention will be described in detail below with reference to the accompanying drawings and examples.
[0067] The present invention first uses modern optimization algorithms to estimate the phase parameters of each order of each component of the polynomial phase signal. The modern optimization algorithms that can be used here mainly include evolutionary algorithms, swarm intelligence algorithms, simulated annealing algorithms, tabu search algorithms, etc. Among them, evolutionary algorithms specifically include genetic algorithm, differential evolution algorithm, immune algorithm, etc.; swarm intelligence algorithms specifically include ant colony algorithm, particle swarm algorithm, etc. The above-mentioned optimization algorithm can be used to realize the phase parameter estimation of each order of the polynomial phase signal, thereby obtaining the phase parameters of each order of each signal component forming the polynomial phase signal, and simultaneous...
PUM
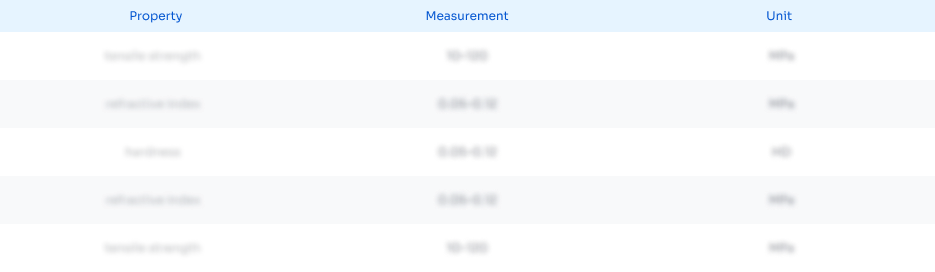
Abstract
Description
Claims
Application Information
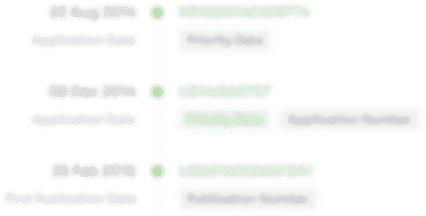
- R&D
- Intellectual Property
- Life Sciences
- Materials
- Tech Scout
- Unparalleled Data Quality
- Higher Quality Content
- 60% Fewer Hallucinations
Browse by: Latest US Patents, China's latest patents, Technical Efficacy Thesaurus, Application Domain, Technology Topic, Popular Technical Reports.
© 2025 PatSnap. All rights reserved.Legal|Privacy policy|Modern Slavery Act Transparency Statement|Sitemap|About US| Contact US: help@patsnap.com