Visual representation learning for brain tumor classification
A brain tumor and classifier technology, applied in the field of learning brain tumor classification, can solve the problem of low classification accuracy
- Summary
- Abstract
- Description
- Claims
- Application Information
AI Technical Summary
Problems solved by technology
Method used
Image
Examples
Embodiment Construction
[0020] Since it is extremely difficult to gain a clear understanding of the visual properties of tumor-affected regions under the current limitations of CLE imaging, a more efficient data-driven visual representation learning strategy is used. An exhaustive set of filters is implicitly learned from the training data, which are used to efficiently represent even slightly similar images. The learned representations are used as input to any classifier without further parameter tuning.
[0021] For many image analysis tasks, the quality of one or more features is important. Useful features can be built from raw data using machine learning. The involvement of machines can distinguish or identify useful features better than humans. Given the large number of possible features of images and the diversity of image sources, machine learning methods are more robust than human programming.
[0022] Provides a network framework for building features from raw image data. The network fra...
PUM
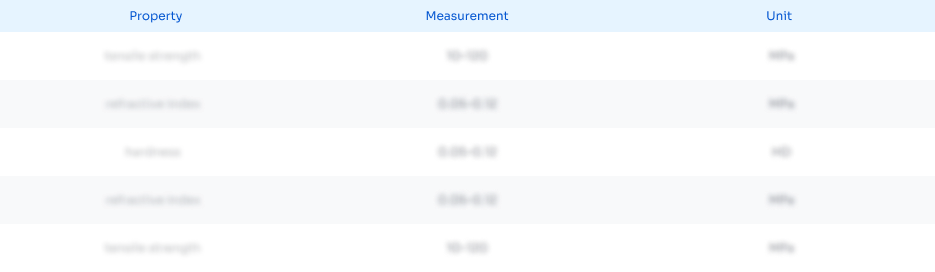
Abstract
Description
Claims
Application Information
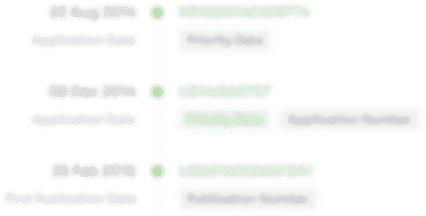
- R&D
- Intellectual Property
- Life Sciences
- Materials
- Tech Scout
- Unparalleled Data Quality
- Higher Quality Content
- 60% Fewer Hallucinations
Browse by: Latest US Patents, China's latest patents, Technical Efficacy Thesaurus, Application Domain, Technology Topic, Popular Technical Reports.
© 2025 PatSnap. All rights reserved.Legal|Privacy policy|Modern Slavery Act Transparency Statement|Sitemap|About US| Contact US: help@patsnap.com