Improved image segmentation training method based on full convolutional neural network
A convolutional neural network and full convolutional network technology, applied in the field of medical image processing, can solve problems such as poor segmentation efficiency, insufficient feature extraction, and large amount of calculation, so as to improve training efficiency, reduce training time, and avoid over-fitting combined effect
- Summary
- Abstract
- Description
- Claims
- Application Information
AI Technical Summary
Problems solved by technology
Method used
Image
Examples
Embodiment Construction
[0020] Such as figure 1 As shown, the present invention constructs an improved segmentation training method based on a full convolutional neural network, and the specific technical details are as follows:
[0021] (1) Collect images of skin surface melanoma and preprocess the images;
[0022] First, use anisotropic diffusion filtering algorithm to denoise the input image, then perform adaptive histogram equalization processing on the denoised image for data enhancement, and rotate, flip, and deform each sample to obtain data The enhanced training samples and validation samples, the sample size is 513×513.
[0023] (2) Training Convolutional Neural Networks for Classification
[0024] The image processed in the first step and the label image are cut and sampled in a size of 64×64 to obtain a cut and sampled image that meets the number of training samples. Then, according to the ratio (75%, 25%) of foreground pixels and background pixels in the cut label image, these sample ...
PUM
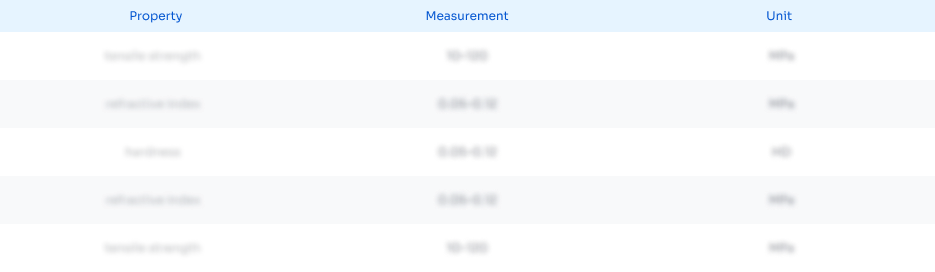
Abstract
Description
Claims
Application Information
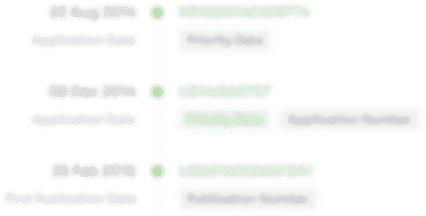
- R&D Engineer
- R&D Manager
- IP Professional
- Industry Leading Data Capabilities
- Powerful AI technology
- Patent DNA Extraction
Browse by: Latest US Patents, China's latest patents, Technical Efficacy Thesaurus, Application Domain, Technology Topic, Popular Technical Reports.
© 2024 PatSnap. All rights reserved.Legal|Privacy policy|Modern Slavery Act Transparency Statement|Sitemap|About US| Contact US: help@patsnap.com