Sparse coding method based on local and global regularization
A sparse coding and global technology, applied in the field of sparse coding, which can solve the problem that low-dimensional representation cannot maintain the local and global geometric structure of data at the same time.
- Summary
- Abstract
- Description
- Claims
- Application Information
AI Technical Summary
Problems solved by technology
Method used
Image
Examples
Embodiment Construction
[0092] The present invention will be further described below in conjunction with the accompanying drawings and embodiments.
[0093] This embodiment proposes a sparse coding method based on local and global regularization. The objective function and solution method of this method are as follows:
[0094] (1) Local and global regularization
[0095] Traditional sparse coding methods cannot capture the inherent geometric structure in the data because they only exploit the local or global structure of the data alone. A reasonable approach is to make full use of the local and global structural information of the data during the representation process.
[0096] A large number of studies have shown that in the data representation, making full use of the local geometric structure of the data can improve the performance of the algorithm. Therefore, the present invention exploits local regression regularization to discover the underlying geometric structure of the data. Specifically...
PUM
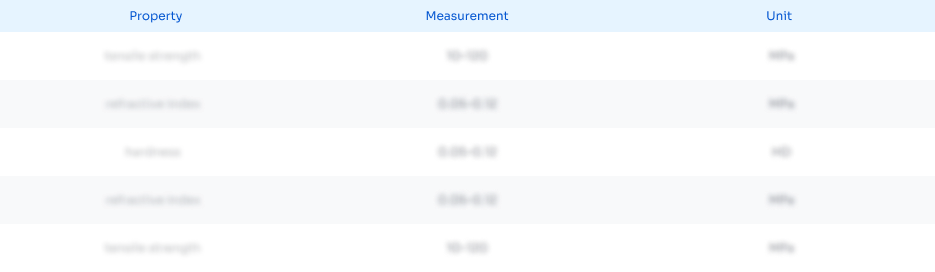
Abstract
Description
Claims
Application Information
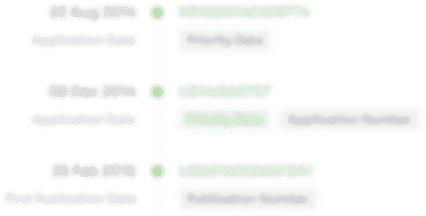
- Generate Ideas
- Intellectual Property
- Life Sciences
- Materials
- Tech Scout
- Unparalleled Data Quality
- Higher Quality Content
- 60% Fewer Hallucinations
Browse by: Latest US Patents, China's latest patents, Technical Efficacy Thesaurus, Application Domain, Technology Topic, Popular Technical Reports.
© 2025 PatSnap. All rights reserved.Legal|Privacy policy|Modern Slavery Act Transparency Statement|Sitemap|About US| Contact US: help@patsnap.com