Partial discharge defect type recognition method and system based on convolutional neural network
A technology of convolutional neural network and defect type, which is applied in the direction of measuring electricity, measuring electrical variables, and testing dielectric strength, etc., can solve the problems of complex partial discharge, large influence of interference, high uncertainty, etc., and achieve reliable recognition results , the effect of reducing the probability of misjudgment
- Summary
- Abstract
- Description
- Claims
- Application Information
AI Technical Summary
Problems solved by technology
Method used
Image
Examples
Embodiment 1
[0040] This embodiment provides a partial discharge defect category recognition method based on a convolutional neural network, such as figure 1 shown, including the following steps:
[0041] A. Collect partial discharge signals with known partial discharge defect types;
[0042] B. Establish a partial discharge information library according to step A, which includes partial discharge waveform signals of several defect types and corresponding defect types;
[0043] The above steps A and B are, for example, using simulated partial discharge sources to simulate partial discharges from tip defects, partial discharges from surface defects, partial discharges from air gap defects and partial discharges from floating defects in power equipment. The pulse current waveform signals of the four defect types were collected using the pulse current method. After collecting 1000 pieces of waveform data for each type of partial discharge, a partial discharge information library including t...
Embodiment 2
[0058] This embodiment provides a partial discharge defect category recognition system based on a convolutional neural network, including:
[0059] Known signal collection unit: collect partial discharge signals with known partial discharge defect types;
[0060] Partial discharge information library establishment unit: establish a partial discharge information library according to the partial discharge signals collected by the known signal acquisition unit, which includes partial discharge waveform signals of several defect types and corresponding defect types;
[0061] Convolutional neural network building unit: build a convolutional neural network for pattern recognition;
[0062] Convolutional neural network training unit: use the partial discharge signal waveform and the corresponding defect type in the partial discharge information database as input to train the convolutional neural network;
[0063] Undetermined signal acquisition unit: collect multiple partial dischar...
PUM
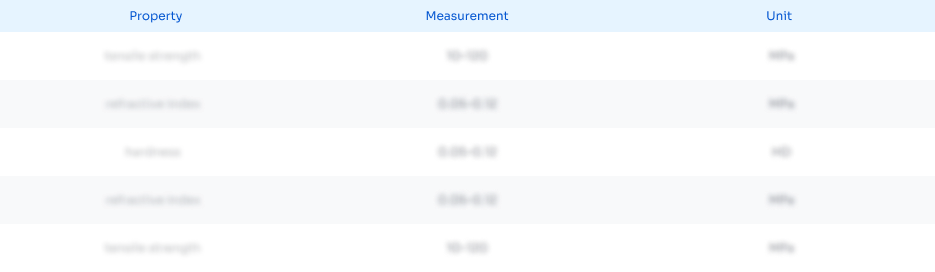
Abstract
Description
Claims
Application Information
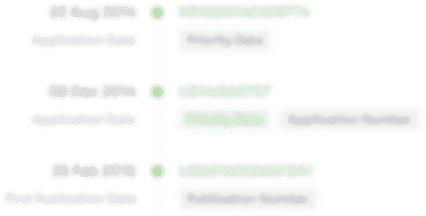
- R&D Engineer
- R&D Manager
- IP Professional
- Industry Leading Data Capabilities
- Powerful AI technology
- Patent DNA Extraction
Browse by: Latest US Patents, China's latest patents, Technical Efficacy Thesaurus, Application Domain, Technology Topic, Popular Technical Reports.
© 2024 PatSnap. All rights reserved.Legal|Privacy policy|Modern Slavery Act Transparency Statement|Sitemap|About US| Contact US: help@patsnap.com