Wind power forecast method based on continuous period clustering
A technology for wind power forecasting and time period, applied in forecasting, instruments, biological neural network models, etc., can solve problems such as difficulty in guaranteeing accuracy, large dependence, and failure to consider the influence of reference power curves and meteorological characteristic values, etc., to achieve improved Accuracy, the effect of improving forecasting precision and accuracy
- Summary
- Abstract
- Description
- Claims
- Application Information
AI Technical Summary
Problems solved by technology
Method used
Image
Examples
Embodiment 1
[0048] Such as figure 1 As shown, direct application of the traditional method based on similar days for wind power prediction is not very effective. If the data is analyzed on a daily basis, the upper power curve will be ignored due to its low similarity to the target power curve, resulting in loss of information. However, after the time period is appropriately shortened, effective information can be found in historical data. The selection of similar period length is very critical. If the time scale is too long, irrelevant data will be introduced, resulting in time-consuming prediction process and reduced accuracy. On the contrary, it cannot reflect the changing trend of the power curve and the potential regularity information.
[0049] Taking a wind farm in Guizhou as the research object, the experimental data is taken from the operation data of a wind farm in Guizhou Province from September 2015 to December 2016, and the weather forecast data comes from NWP.
[0050] Com...
PUM
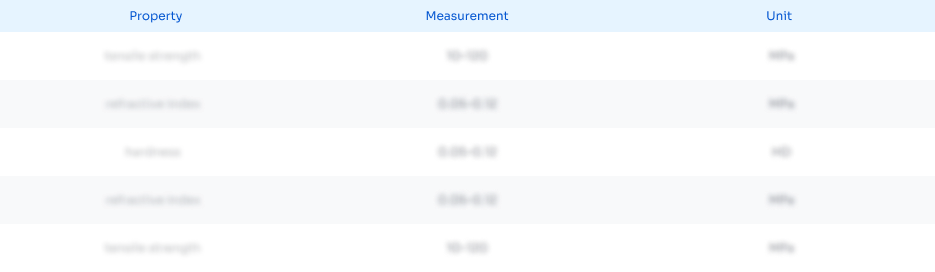
Abstract
Description
Claims
Application Information
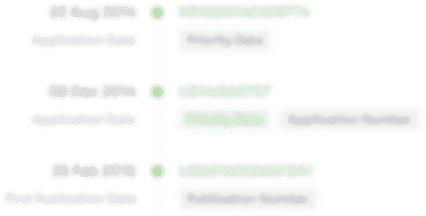
- R&D
- Intellectual Property
- Life Sciences
- Materials
- Tech Scout
- Unparalleled Data Quality
- Higher Quality Content
- 60% Fewer Hallucinations
Browse by: Latest US Patents, China's latest patents, Technical Efficacy Thesaurus, Application Domain, Technology Topic, Popular Technical Reports.
© 2025 PatSnap. All rights reserved.Legal|Privacy policy|Modern Slavery Act Transparency Statement|Sitemap|About US| Contact US: help@patsnap.com