Novel transductive semi-supervised data classification method and system
A data classification and semi-supervised technology, applied in character and pattern recognition, instruments, computer components, etc., can solve the problem of high data label overhead
- Summary
- Abstract
- Description
- Claims
- Application Information
AI Technical Summary
Problems solved by technology
Method used
Image
Examples
Embodiment 1
[0030] The present invention was tested on three data sets of UCI machine learning database: Ionosphere and Balacce scale and SCCTS. Among them, Ionosphere contains 351 categories and 34 attributes; Balacce scale contains 132 samples and 3 categories; SCCTS contains 600 samples and 6 categories. Each set of experiments selects 1 to 9 training samples from each database in turn, and observes the classification accuracy. These databases are collected from multiple sources so that the test results are generally descriptive.
[0031] Please refer to the attached figure 1 , is a flow chart of a new transductive semi-supervised data classification method disclosed in the embodiment of the present invention, and the specific implementation steps are:
[0032] A new transductive approach to semi-supervised data classification, consisting of:
[0033](1), preprocess the original data set, randomly divide the original data set into a labeled training set and an unlabeled training set...
PUM
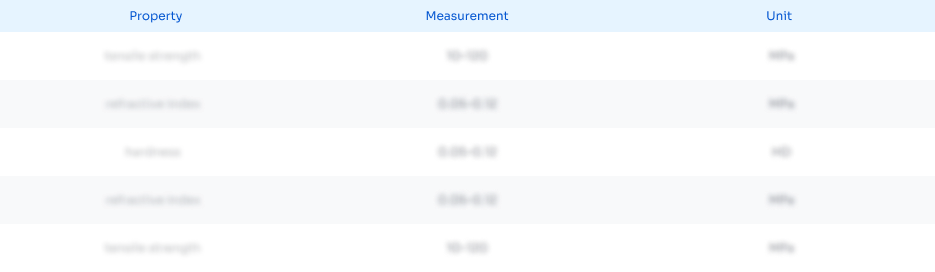
Abstract
Description
Claims
Application Information
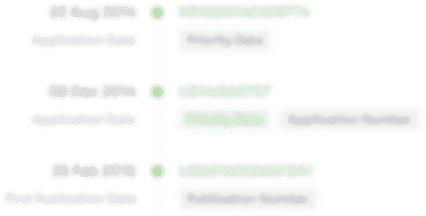
- Generate Ideas
- Intellectual Property
- Life Sciences
- Materials
- Tech Scout
- Unparalleled Data Quality
- Higher Quality Content
- 60% Fewer Hallucinations
Browse by: Latest US Patents, China's latest patents, Technical Efficacy Thesaurus, Application Domain, Technology Topic, Popular Technical Reports.
© 2025 PatSnap. All rights reserved.Legal|Privacy policy|Modern Slavery Act Transparency Statement|Sitemap|About US| Contact US: help@patsnap.com