Accelerator beam track control method and system based on depth reinforcement learning
A technology of reinforcement learning and accelerators, applied in general control systems, control/regulation systems, adaptive control, etc., can solve problems such as labor-intensive and time-consuming, PID tuning parameters rely on engineering experience, and complex beam trajectory control problems
- Summary
- Abstract
- Description
- Claims
- Application Information
AI Technical Summary
Problems solved by technology
Method used
Image
Examples
Embodiment Construction
[0091] In order to make the object, technical solution and advantages of the present invention clearer, the present invention will be further described in detail below in conjunction with specific embodiments and with reference to the accompanying drawings.
[0092] The invention discloses a method and system for controlling the beam trajectory of an accelerator based on deep reinforcement learning, which is used to control the beam trajectory of the accelerator in a target state. The method utilizes training data and adopts a deep reinforcement learning method to perform a deep neural network Pre-training, store the weight parameters of the trained deep neural network and the empirical data of the orbit control strategy; use the beam position monitor to obtain the state data of the beam orbit online, feed it into the deep neural network, and The output of the deep neural network is coupled to the beam track corrector; the weight data of the trained deep neural network and the ...
PUM
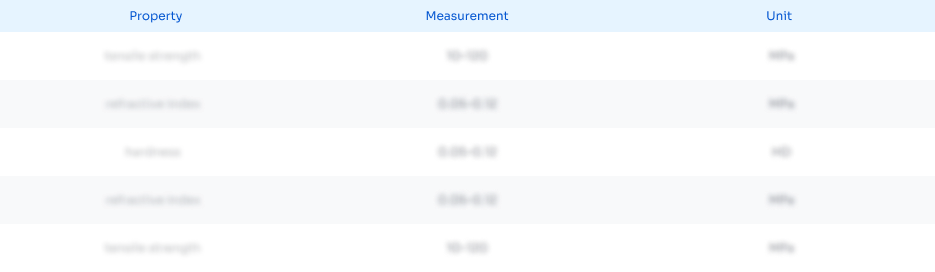
Abstract
Description
Claims
Application Information
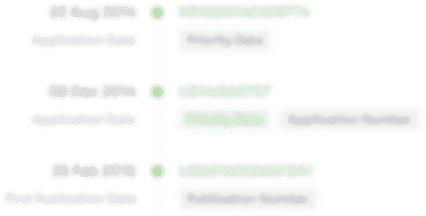
- R&D Engineer
- R&D Manager
- IP Professional
- Industry Leading Data Capabilities
- Powerful AI technology
- Patent DNA Extraction
Browse by: Latest US Patents, China's latest patents, Technical Efficacy Thesaurus, Application Domain, Technology Topic.
© 2024 PatSnap. All rights reserved.Legal|Privacy policy|Modern Slavery Act Transparency Statement|Sitemap