Mean influence value data transformation-based k-nearest neighbor fault diagnosis method
A technology of average influence value and k-nearest neighbor, applied in the field of fault diagnosis, can solve problems such as difficulty in obtaining prior information, difficulty in feature extraction, and low recognition rate of principal component analysis algorithm, so as to enhance correlation and reduce modeling complexity. , the effect of reducing the dimension
- Summary
- Abstract
- Description
- Claims
- Application Information
AI Technical Summary
Problems solved by technology
Method used
Image
Examples
Embodiment Construction
[0043] The following will clearly and completely describe the technical solutions in the embodiments of the present invention with reference to the accompanying drawings in the embodiments of the present invention. Obviously, the described embodiments are only some, not all, embodiments of the present invention. Based on the embodiments of the present invention, all other embodiments obtained by persons of ordinary skill in the art without making creative efforts belong to the protection scope of the present invention.
[0044] A k-nearest neighbor fault diagnosis method based on average influence value data transformation, the steps are as follows:
[0045] S1, collecting a data set X, the data set X includes L data sets x(k);
[0046] X=[x(1),x(2),...,x(L)](1);
[0047] x(k)=[x 1 (k),x 2 (k),...,x i (k),...,x n (k)] T (2);
[0048] Among them, X is the data set, x(k) is the kth data set in the data set, k=1,2,...,L; x i (k) is the i-th data in the k-th data set, i=1...
PUM
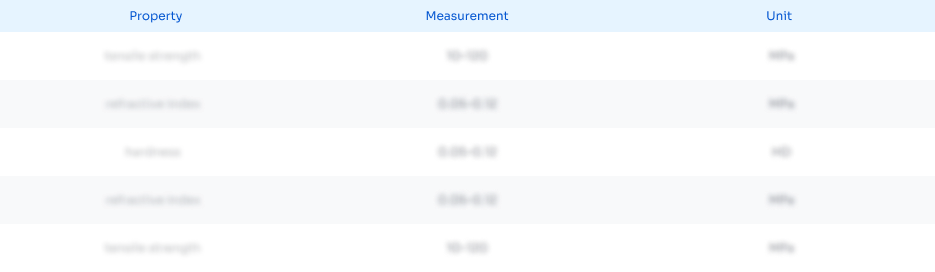
Abstract
Description
Claims
Application Information
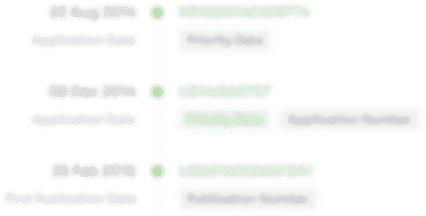
- R&D Engineer
- R&D Manager
- IP Professional
- Industry Leading Data Capabilities
- Powerful AI technology
- Patent DNA Extraction
Browse by: Latest US Patents, China's latest patents, Technical Efficacy Thesaurus, Application Domain, Technology Topic, Popular Technical Reports.
© 2024 PatSnap. All rights reserved.Legal|Privacy policy|Modern Slavery Act Transparency Statement|Sitemap|About US| Contact US: help@patsnap.com