Face recognition method based on convolutional neural network
A convolutional neural network, face recognition technology, applied in character and pattern recognition, instruments, computer parts and other directions, can solve the problems of poor self-adaptation, low accuracy, etc., to achieve enhanced robustness, short time cost, The effect of reducing complexity
- Summary
- Abstract
- Description
- Claims
- Application Information
AI Technical Summary
Problems solved by technology
Method used
Image
Examples
specific Embodiment approach 1
[0021] Specific implementation mode one: as figure 1 As shown, a face recognition method based on convolutional neural network includes the following steps:
[0022] Step 1: Divide the face images to be recognized into three categories: training samples, test samples and verification samples, and read the data to be trained, each of which has its corresponding label;
[0023] Step 2: Normalize the face image after reading the training data in step 1;
[0024] Normalize the read face image, the reason is that the neural network is trained (probability calculation) and predicted by the statistical probability of the sample in the event, and the normalization is between 0-1 Statistical probability distribution, when the input signals of all samples are positive, the weights connected to the neurons of the first hidden layer can only increase or decrease at the same time, resulting in a very slow learning speed. In order to avoid this situation and speed up the network learning ...
specific Embodiment approach 2
[0029] Specific embodiment two: the difference between this embodiment and specific embodiment one is: the structure of the convolutional neural network is input layer-convolution layer-convolution layer-pooling layer-convolution layer-convolution layer-pool Layer-full connection layer arrangement, because the gradient of the ReLu function is not saturated and the calculation speed is fast, so the function converges faster, so we choose the ReLu function as the activation function, and the pooling method uses the maximum pooling method, such as figure 2 As shown, the output of the current layer is expressed as:
[0030] x e =f(u e )
[0031] u e =W e x e-1 +b e
[0032] where x e Indicates the output of the current layer, u e Represents the input of the activation function (the result after calculating the weight and bias of the current layer), f() represents the activation function, W e is the weight of the current layer, b e can be biased.
[0033] Other steps a...
specific Embodiment approach 3
[0034] Embodiment 3: The difference between this embodiment and Embodiment 1 or 2 is that in Step 3, Dropout is added after the pooling layer to disconnect the network, and the neurons are disconnected with a probability of 0.25 and 0.5. Connection.
[0035] Such as image 3 with Figure 4 As shown, the Dropout layer can randomly disconnect the connection of the network, which can effectively suppress the phenomenon of overfitting. Since the number of disconnected connections should not be too many, too few will also affect the effect, so we choose to disconnect the connections between neurons with the probability of 0.25 and 0.5.
[0036] Other steps and parameters are the same as those in Embodiment 1 or Embodiment 2.
PUM
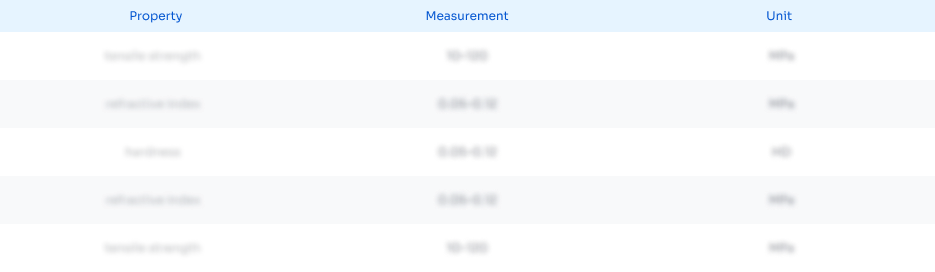
Abstract
Description
Claims
Application Information
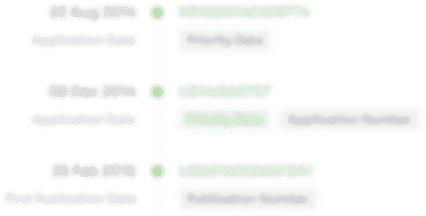
- R&D Engineer
- R&D Manager
- IP Professional
- Industry Leading Data Capabilities
- Powerful AI technology
- Patent DNA Extraction
Browse by: Latest US Patents, China's latest patents, Technical Efficacy Thesaurus, Application Domain, Technology Topic, Popular Technical Reports.
© 2024 PatSnap. All rights reserved.Legal|Privacy policy|Modern Slavery Act Transparency Statement|Sitemap|About US| Contact US: help@patsnap.com