A No-Reference Image Quality Objective Evaluation Method Based on Multi-Scale Generative Adversarial Network
An objective quality evaluation and reference image technology, applied in image analysis, image enhancement, image data processing, etc., can solve the problems of no reference, poor performance of image quality evaluation without reference, etc., and achieve the effect of excellent performance
- Summary
- Abstract
- Description
- Claims
- Application Information
AI Technical Summary
Problems solved by technology
Method used
Image
Examples
Embodiment approach
[0036] The flow chart of the implementation is as figure 1 shown, including the following steps:
[0037] Step S10, generating a similar quality image library;
[0038] Step S20, training a multi-scale generative adversarial network for similar quality graphs;
[0039] Step S30, training the quality score regression network;
[0040] Step S40, performing no-reference quality evaluation on the distorted image.
[0041] The step S20 of training similar quality map multi-scale generative confrontation network adjustment step S20 of the embodiment also includes the following steps:
[0042] In step S200, each distorted image in the TID2013 database and its corresponding GMSD similar quality image are subjected to sliding windows of different sizes for three times, and the sizes of the sliding windows are 96×96, 144×144, and 194×194, respectively, to generate three copies of one-to-one correspondence The color distortion image block is similar to the grayscale image block;
[...
PUM
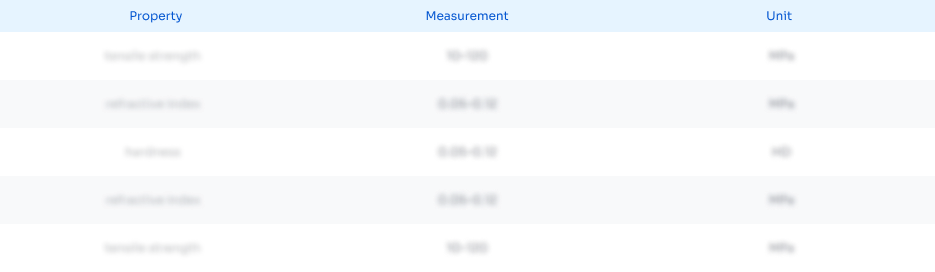
Abstract
Description
Claims
Application Information
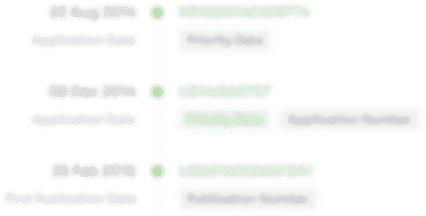
- R&D Engineer
- R&D Manager
- IP Professional
- Industry Leading Data Capabilities
- Powerful AI technology
- Patent DNA Extraction
Browse by: Latest US Patents, China's latest patents, Technical Efficacy Thesaurus, Application Domain, Technology Topic, Popular Technical Reports.
© 2024 PatSnap. All rights reserved.Legal|Privacy policy|Modern Slavery Act Transparency Statement|Sitemap|About US| Contact US: help@patsnap.com